bias, or unfairness, is omnipresent in society, and is becoming more debated now that it has become clear that not only humans, but also algorithms are biased in their decision-making. These non-rational judgements can have a great negative impact on people’s lives, for example when negatively biased against certain demographic groups, a specific gender, race, or sexual orientation. Countless instances have been discussed where a biased algorithm inflicted damage upon certain individuals or groups, such as an algorithm assessing risk that was discriminating against black prisoners in a US court which was revealed in a report by Propublica. How shall we deal with these discoveries? Is the solution to solemnly rely on human judgement again? We argue that biases that occur in algorithms are (partly) a representation of human biases since we are the ones creating them and feeding them with (potentially biased) data. One cannot “rewire” emotional humans to get rid of their biases, but one can adapt a more rational artificial intelligence in such a way that the impact of unfair judgements is minimized. Thus, we argue that human biases are more problematic than the ones found in judgements made by algorithms.
Of course, there is an immense variety of types of biases, as well as artificial intelligence (AI) applications where they could have an impact. To give a small illustration, if you do a quick Google Image search on “executive”, or “manager” you mostly find them depicted by white males. Now, it would be easy to argue that Google’s algorithm is biased when selecting which images to show us, however, the algorithm just selects the images that are most frequently available online. Of course, we do not agree with representing society this way, even if these are the most frequently available pictures. We disagree, however, with the thought that this is a problem which is caused by and unique to artificial intelligence. To this end, AI is not a natural phenomenon, but man-made. Humans, like Google’s algorithm, also tend to show an availability bias, causing them to overestimate how likely a certain event is, based on how readily available it is in memory. Put simply, humans will also tend to imagine more often a white male when thinking about an executive or a manager since we retrieve from memory what occurs most often and thus what our society currently looks like.
Human Bias
Biases in humans emerge from a very young age, because of the limited insight and information that humans have about the world. Instead of thinking of all the people who could possibly be managers of a company, we apply heuristics and select the instances that are most frequently and readily available in our memory (i.e., availability bias). Instead of looking at the females that we encounter in management positions, we will often focus more on the evidence showing that managers are predominantly male, since it confirms our beliefs (i.e., confirmation bias). Of course, these are just oversimplified examples that may or may not apply to you, but these and many more biases are present in humans, in more or less quantity, to make it easier to judge individuals and situations when there is limited time to do so. Some more examples of biases and their (short) descriptions can be found in Table 1.
Cognitive bias | Description |
---|---|
Availability Bias | Tendency to base a certain likelihood to the information that is most frequently or most readily available in memory |
Confirmation Bias | Tendency to focus on information that is in line with what one believes, while ignoring information that contradicts these believes |
Halo Effect | Tendency to generalize a person’s positive traits or (personality) characteristics to other (personality) characteristics of this person; opposite of a “Reversed Halo” |
Fundamental Attribution Error | Tendency to attribute others’ actions more to their personality than to situational factors |
Hindsight bias | Tendency to believe that certain outcomes could or should have anticipated, even though certain information was not available yet |
Negativity bias | Tendency to put more (negative) value on losing, than (positive) value on winning, making humans weight negative outcomes stronger than positive ones |
AI Bias
Even though we expect algorithms to be fair and rational when making judgements, as has and will be discussed throughout this article, algorithms are also often biased. The biases that AI systems inherit can be divided into roughly two categories. First of all, there are cognitive biases, which are the ones that cause people to be misjudged based on group membership (e.g., being female). These get transferred into the algorithm either by (unconsciously) introducing them directly into the model, or by using a biased dataset to train the algorithm. Second, we have biases due to incomplete data. If the model is trained on an incomplete dataset, that does not contain a true representation of the population, it will not know how to handle the data of certain groups, which can lead to bias or misjudgement.
If the model is trained on an incomplete dataset, it will not know how to handle the data of certain groups, which can lead to bias or misjudgement.
An example of a cognitive bias is the AMZN.O machine learning algorithm. The recruiting tool sorted the resumes of all applicants, aiming to find the most talented candidates. The algorithm, however, seemed to have a preference for men over women, not because of their gender itself. AMZN.O favored resumes with words like “executed” or “captured”, which are used more often by male than by female applicants, while it penalized resumes with the word “women’s” (e.g., “women’s college”) on them. Fortunately, these problems can be detected early on as was the case here. An algorithm is tested extensively on a diverse range of datasets making it possible to shut the system down if needed and to possibly re-train it in order to overcome detected biases.
Humans vs AI: Who is less biased?
Real-life case
Of course, examples of big companies where an algorithm did not remove human biases, but instead automated or even accelerated them can be a scary thought. How could less sophisticated companies accomplish biased-free algorithms if even Amazon was not able to generate a fair selection algorithm? We need to keep in mind that not only the amount that companies invest in AI, but also the amount of ongoing research is increasing at an exponential rate. The Amazon algorithm was launched around seven years ago and much research has been done since. Thus, we can expect many improvements when developing similar algorithms, that is if companies are actually putting enough effort into it.
An older case where a university hired applicants based on a computer algorithm seemed to show a similar bias as the ones explained previously. Applicants with non-European names, as well as women, were discriminated against by the algorithm. This shows that the problem of biased AI is already rather old, but at the same time we have to keep in mind that we also (often) find biased judgements made by humans in similar situations.
So, should we go back to exclusively human decision making?
As outlined above, AI can have a significant effect on people’s lives. It is increasingly used across multiple sectors including i.e. the financial sector or the government and the technology further develops at a rapid pace. Almost too fast to keep up formulating laws and guidelines that control the structure and content of the algorithms. AI technologies are still in the development phase and one could argue that until there are no clear laws AI systems should not be applied in the real world, but only researched in laboratories. Realistically speaking, this will not be enforceable anymore and what we instead need are new laws to control these fairly new technologies. The European Union, for example, passed the General Data Protection Legislation in 2016. Although the interpretation of the law might still be partly ambiguous it is a first step towards formulating new laws that can be further adjusted if needed. Also companies increasingly try to stay ahead of the legislation and show increasing attempts to adhere to the ethical guidelines and laws.
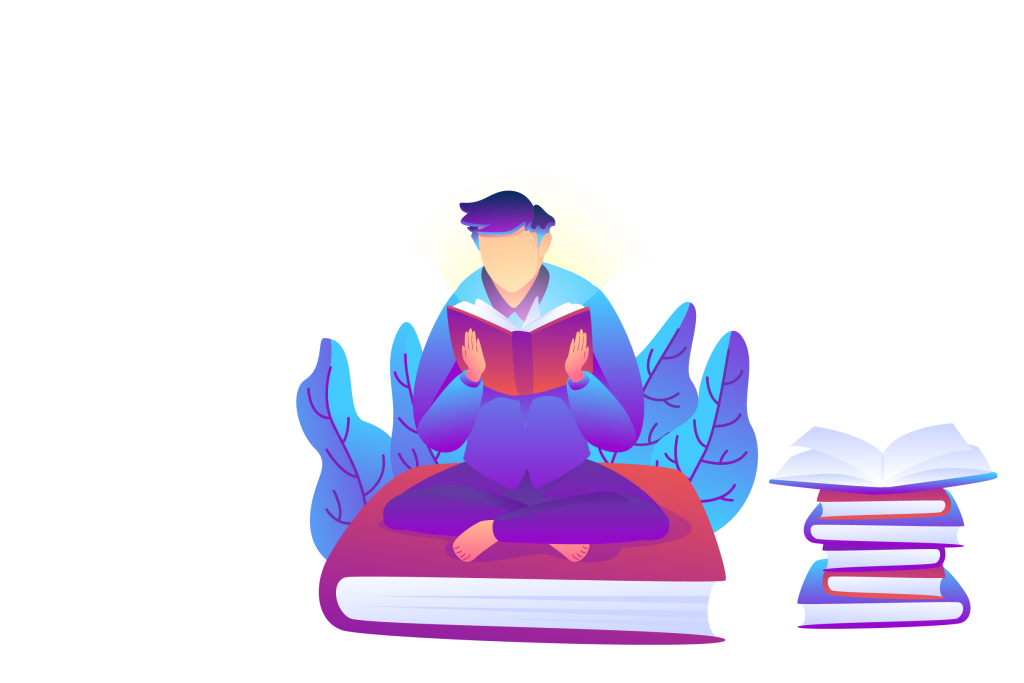
Humans try to be rational, but will always remain, at least in part, emotional decision-makers
These new laws are also needed to prevent AI systems being used in a (fraudulent) way that was initially not intended or stated. One famous example of such misuse of data is the scandal of Cambridge Analytica/SCL. The company used data of facebook users to influence their voting behavior, amongst others in the Trump campaign 2016 and the Brexit campaign. Former director of business development at Cambridge Analytica, Brittney Kaiser, even went as far as calling the techniques used by the company ‘weapons-grade communications’ tactics that were used against the UK population during the successful Brexit campaign. Today, Kaiser is an advocate for data rights, supports legislators to pass privacy laws and pleads for more regulation of big tech firms like Facebook to prevent algorithms being used for bad. This example illustrates the need for more laws and in case of a misuse of data that not only the collected data is deleted, but also the algorithms they were fed into.
Most of us perceive machines and with that AI as more objective than us humans, which could make us believe the judgement generated by an AI more easily than that of a human and potentially make us more blind towards biases caused by AI. In order to forecome this, we need training that helps us to spot biases in algorithms e.g. when applied to recruitment processes. Trained people should then be able to recognize biases caused by AI. While this might still be a challenging task it is presumably easier than recognizing human biases because of our rather ambiguous, inconsistent and potentially (if algorithms are improved) less rational decision-making processes.
There might also be some voices that simply claim that decisions for humans should be made by humans. Outsourcing decisions to a machine is a new phenomenon and thus might be as scary as it can be exhilarating. Studies show that we judge mistakes and biases made by AIs more harshly than our own, i.e. we might also be biased against machines. However, they do bring a number of advantages with them. For one, we can analyze the decisions made by an algorithm post hoc. It is not an easy task to keep track of all the potential biases, but compared to human biases the ones implemented in AI are controllable and can thus be eliminated on a long-term basis. It is unlikely the same accounts for humans.
Reducing human biases is an extremely complex process, since these have emerged over countless years, and are “hardcoded” in our brains, in more or less quantity depending on the individual. Biases have evolved as adaptive algorithms in our brains, making us use prior experiences to decide on current actions. We can try to learn about biases, try to recognize them in ourselves and others, and try to go against our intuitions to ensure that we become less biased in our decisions. However, since human beings are emotional rather than purely rational, it will be extremely hard if not impossible to fully get rid of biases, even if we try.
Humans need to rely on heuristics, which are “hardcoded” into our brains, among others because of our working memory limitations.
One of the challenges that human judgement implies is that it often is biased while at the same time it appears not to be. This makes it difficult to detect human biases in the somewhat ambiguous process of human decision making. We know that humans are not fully rational, and make errors of judgement, however, it is extremely hard to try to overcome these biases, both on an individual level, as well as recognizing them in other people. The difficulty of detecting biases in humans makes it a complex problem to solve, which might lead one to think that biases derived from AI systems are less problematic.
Humans’ decisions are not consistent. Given the same situation it is likely that two people will come to two different conclusions. Nobel Prize winner Daniel Kahnemann addressed this in his book ‘Thinking, fast and slow’ and amongst others cognitive illusions. According to him, there is not much hope that we can overcome these illusions. Comparable to optical illusions (see figure) it is merely impossible for us to see that the two lines are actually of the same length even if we know this to be the truth. With that in mind, it is substantially more difficult to control human biases than biases caused by AIs. We cannot “rewire” human decision-making like we can do with an algorithm. This means that if we can create a more objective alternative, possibly an algorithm, it would not be recommended to go back to exclusively human decision making

Inspired by: Yagoda, B. (2018). The cognitive biases tricking your brain, The Atlantic.
What can AI mean for the future of decision making?
The previous paragraph illustrates that returning exclusively human decision making is not likely, nor desirable. Instead of focusing on what goes wrong in AI judgements we should lay our focus on how to detect and solve biases to make AI systems more rational in the future. Solving the biases that AI-algorithms can show relies on a few different steps as suggested by different sources, such as the Harvard Business review and the McKinsey Global Institute (see image). If we apply these solutions in a systematic manner, the amount of bias in algorithms could be decreased, in turn increasing the trust that people have in AI.
Inspired by: Harvard Business Review and McKinsey
By detecting possible biases in humans, as well as in AI, it becomes easier for companies to detect biased algorithms in an early stage. This can be converted into a plan of action, where it is decided beforehand what judgement errors could emerge, how these can be found (i.e., what exactly to test), and how humans can work together with AI to prevent them from happening again. Plans of action, or debiasing strategies, are out of the scope of this article, but are mainly based on identification of potential sources of bias, data collection processes, as well as establishing transparent metrics. The interested reader can consult some of these strategies at the Google AI best practices. To make this all possible, it is important that companies keep investing in decent research, as well as in diversifying the field of AI and making it more multi-disciplinary.
Apart from being more sustainably objective, what does a future, rational algorithm add to the judgement of trained humans like lawyers or human resources managers who we expect to be logical and rational? Humans can never take into account all aspects of a situation before they make a judgement, since it exceeds memory capacities. This not only makes it impossible for humans to be fully rational forcing them to rely on (non-ethical) heuristics, but also means that AI can make decisions much faster. Algorithms can, if the explained guidelines are followed, be the future of fast, reliable, and objective decision making. However, there is still a lot of work to be done in order to eliminate biases that algorithms inherit from their developers and training data.
Conclusion
As explained, human biases are not only hard to detect, but even harder to solve. Humans need to rely on heuristics, which are “hardcoded” into our brains, among others because of memory limitations, a problem that computers do not face. In the short term, we should still be cautious with the use of AI when making important decisions, first because additional measures such as new laws and guidelines are needed, and second because there are still too many occasions in which algorithms have been shown to copy or even expand the biases of their developers or training data. In addition, it is important to create AI systems for which we can have a better understanding of how they came to their conclusion in order to detect and solve potential biases more efficiently and effectively. This makes it plausible that in the long term human biases will be far more dangerous than AI biases because of our inherently emotional instead of rational nature.

For now, implement hybrid intelligence: humans reviewing the decisions made by algorithms
For now, considering the state-of-the-art decision-making algorithms the solution does not lie in going back to human decision-making but neither in only letting AIs make important decisions. A lot of time can be saved by implementing hybrid intelligence with humans reviewing the decisions made by algorithms as compared to humans making decisions on their own using their limited working-memory capacities. At the same time this can help to detect biases in AI judgements early on and gives us the chance to improve them to eventually end up with (almost) completely rational and logical decision-makers. Instead of focusing on the shortcomings of existing algorithms, we should focus on cooperating with them in order to detect and eliminate misjudgements. Together with rational AI-systems we can aim to create a future free from decision-making based on gender, ethnicity, or other arbitrary factors and to give room to a new era of decision making.