The energy footprint of training and running AI models is a drop in the ocean compared to what these models make possible for the major environmental benefits now, and in the future. We believe we should be training more models rather than handicapping development.
A subject of much debate is the increased environmental impact of digital technology with such immense technological advancement in the digital age. Sitting right at the centre of this argument is Artificial Intelligence (AI) which comes with a substantial need for data and computation. Of course, models such as GPT-4 are absolutely power hungry in terms of training and operating, but this by no means implies that the cost of AI will outweigh its gigantic potential for environmental benefit. This op-ed examines the statistics behind AI’s energy consumption and its role as a crucial tool helping us defend against climate change and environmental degradation. It also argues that putting surcharges on energy consumption for AI companies will act to stop the positive changes that we believe AI will bring, especially at this nascent stage of AI development.
AI Energy Demand
Some recent estimates place daily ChatGPT inference costs at 1GWh, while training a model such as GPT-4 costs around 50GWh. To put that into scale, one single GWh is about what it takes to power 30,000 American households for a day. Although this figure appears somewhat scary when represented this way, this is not so significant compared to the energy consumption of other giants of the Internet and industry – Google Search, cryptocurrency mining, and even the traditional banking sector. For example, daily ChatGPT energy usage is 273x less than cryptocurrency mining, while providing arguably much more benefit for society.
The computational power required by artificial intelligence is rapidly increasing, with a doubling rate of every 3.4 months in terms of petaflops-days. This growth far surpasses the historical trends seen in transistor development, known as Moore’s Law. However, this surge in computational requirements has also led to an increase in the energy demands of AI technologies, prompting the need for innovation in order to decrease their carbon footprint. One solution is the adoption and development of analogue chips, which offers a 14x energy reduction in the AI training stage as compared to traditional TPUs or GPUs. This move towards more energy-efficient technologies is critical for developing greener AI, and is happening without current government intervention.
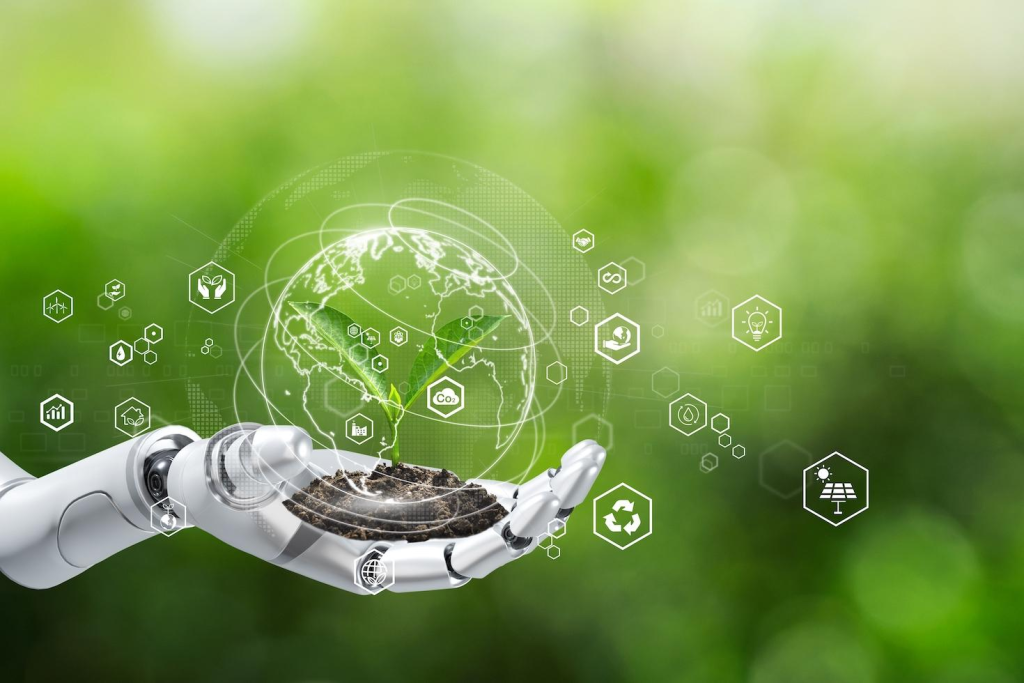
AI’s Green Revolution
AI is not only becoming greener in itself, but it can make any industry it is employed in greener as well. The main focus of this is energy optimisation in tasks such as waste management, power generation, water management, traffic management and general energy reduction across all tasks. According to reports from institutions such as PwC and BCG, AI has the potential of reducing global energy usage by 4-10% by 2030 as better operational efficiency is achieved in many industries. If this research can be trusted, it would not take long for company operations to catch up, any profit driven company would love to reduce their energy bill by 10% without any negatives.
A project from Google DeepMind shows how AI could help the environment by significantly reducing energy consumption required to cool its data centres through system optimisation using AI. The effect of this can be seen in the image below, showing how the project has decreased data centre cooling costs by 40% in terms of power usage effectiveness. This demonstrates that AI can significantly mitigate carbon emissions and this project serves as a blueprint for other industries in reducing their environmental impact.
DeepMind AI Reduces Google Data Centre Cooling Bill by 40% (PUE: Power Usage Effectiveness)
Another example from DeepMind, explores wind predictions, which increases the energy produced by wind turbines by over 20%. These examples show us how AI can improve our industries to be more energy efficient.
Transformational Impacts on Environment by AI
Beyond its current usages, where it is optimising many key areas, AI holds the potential to tackle even more complex environmental challenges, by helping us develop new strategies to deal with a whole assortment of challenges we currently accept as too problematic to completely solve.
Advanced Climate Prediction Models: AI can improve the accuracy and granularity of climate forecasting models, allowing us to prepare for and mitigate climate change impacts. By analysing datasets from weather stations, satellites, and climate models, AI can identify patterns and predict changes better than current algorithms, helping us plan for extreme weather events and long-term climate shifts.
Biodiversity Conservation: By making environmental data accessible and interpretable through complex analysis, AI will be used to protect ecosystems and the unique biodiversity they support.
Solid and Water Waste Management: AI-driven systems are revolutionising resource management approaches, leading to more sustainable practices in handling solid and water waste.
Smart Cities and Renewable Energy: The integration of AI into smart cities and smart grids is reducing energy consumption and promoting the use of renewable energy sources.
Precision Agriculture: AI can optimise farming practices through precision agriculture, which involves using AI algorithms to analyse data from satellite images, drones, and sensors in the field. This technology can help in monitoring crop health, increasing and predicting yields, optimising water usage, and reducing the need for fertilisers and pesticides, minimising environmental impact.
Energy Efficiency in Buildings: AI can be used to manage energy consumption in buildings. By predicting patterns of usage and using external factors like weather conditions, AI systems can adjust heating, ventilation, air conditioning, lighting, and other energy-consuming processes in real-time to minimise waste, and decrease buildings’ carbon footprint.
These advancements prove AI’s abilities to address a wide range of environmental issues, making it a critical tool for sustainable development.
Reasonable concerns
There are, undoubtedly, reasonable concerns moving forward. One significant issue is that certain areas of AI, notably image generation AIs – such as DALL-E, Stable Diffusion, and Midjourney, are quite energy intensive and offer minimal environmental benefits.
In response to these challenges, we believe the future lies in the development of more environment-specific and optimisation-focused AI models. Investments should target areas involving smaller, more self-contained models rather than continuing the current focus on large, data-intensive generative models. While generalised models are beneficial across a wide range of domains and can contribute towards our long-term environmental goals, smaller, more efficient models promise to achieve similar outcomes with reduced energy consumption and greater attentiveness to environmental impact.
In addition to the specific concerns about generative AI, there are valid concerns regarding the environmental impact of data centres that power AI, due to their significant energy consumption. Critics might argue that AI’s reliance on large datasets is inevitably linked, suggesting that discussions about AI’s environmental effects must include the considerations of data storage and processing. However, it’s important to note that the massive increase in stored data predates the recent surge in large language models (LLMs) and deep learning, and will remain a necessity beyond AI applications. Therefore, we state that while the environmental implications of data storage are significant, they should not be solely connected to the advancement of AI. Rather, these issues should be addressed as part of a broader conversation on sustainable data management practices.
AI’s early stages
Although we do admit that AI has some challenges to face when it comes to its environmental impact, we think that as it is in its infancy, we should not add more challenges for startups/research institutions to join the industry, and add valuable insight. Proposed suggestions such as fines, or electricity surcharges for AI companies seem to have their interests not in line with environmental considerations. This will allow only the biggest, most financially powerful companies to compete in the AI field, giving them even more of a monopoly in an industry that is already dominated by big tech resources. Lowering the barrier of entry for smaller startups and research institutions will make it more likely that technology that betters the environment and helps humanity will be developed, and that is why we believe government regulation in the energy usage of AI should be delayed until industry maturity. Currently we should allow as many companies as possible the ability to run their own models, do their research and build potentially environment-saving products.
Conclusion
The debate surrounding the environmental impact of AI must be balanced with an understanding of its potential for sustainable development. We acknowledge the energy demands of training and inferring from AI models, but the benefits these technologies offer in optimisation and new developments vastly outweigh this. The advancements in AI will make industries more efficient and less wasteful but also should be able to tackle some of the most pressing environmental challenges of our times, even before we reach AGI. Furthermore, we are already seeing the development of energy-efficient AI technologies and applications that directly contribute to environmental sustainability. This makes it clear that blocking AI development with premature regulation could hinder progress, and slow down the good work currently being done. Therefore, fostering innovation and supporting the growth of AI in its early stages is important for unlocking its potential to benefit the environment. Encouraging the development of both large-scale generative models and smaller, optimisation-focused AI systems will be the key to making AI sustainable. As AI continues to evolve, it may be one of our strongest tools for environmental conservation, making it crucial to support its development while staying aware of its environmental impact and potential.