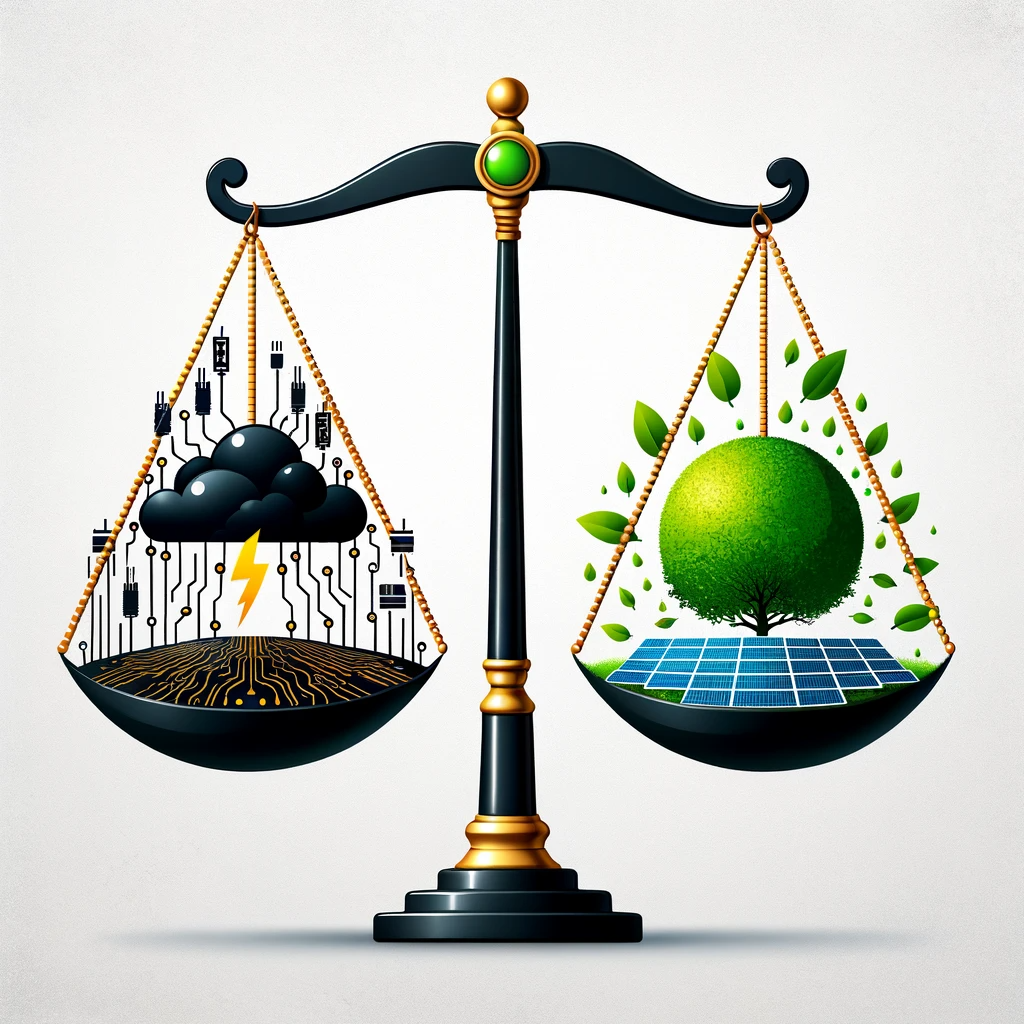
The (Potential) Opportunity
Improving energy efficiency in various industries, assisting the renewable energy grid management, allowing intelligent waste recycling, and assisting environmental policy-making. All of these ways of mitigating the climate crisis, and many more, are being made possible thanks to the increased capabilities of artificial intelligence (AI).
Various academics (for example, see Cowls et al., 2023), and organizations, including the UN, see AI as a potential tool to enhance and broaden our current comprehension of climate change, and it can assist us in developing more efficient solutions to our sustainability problems.
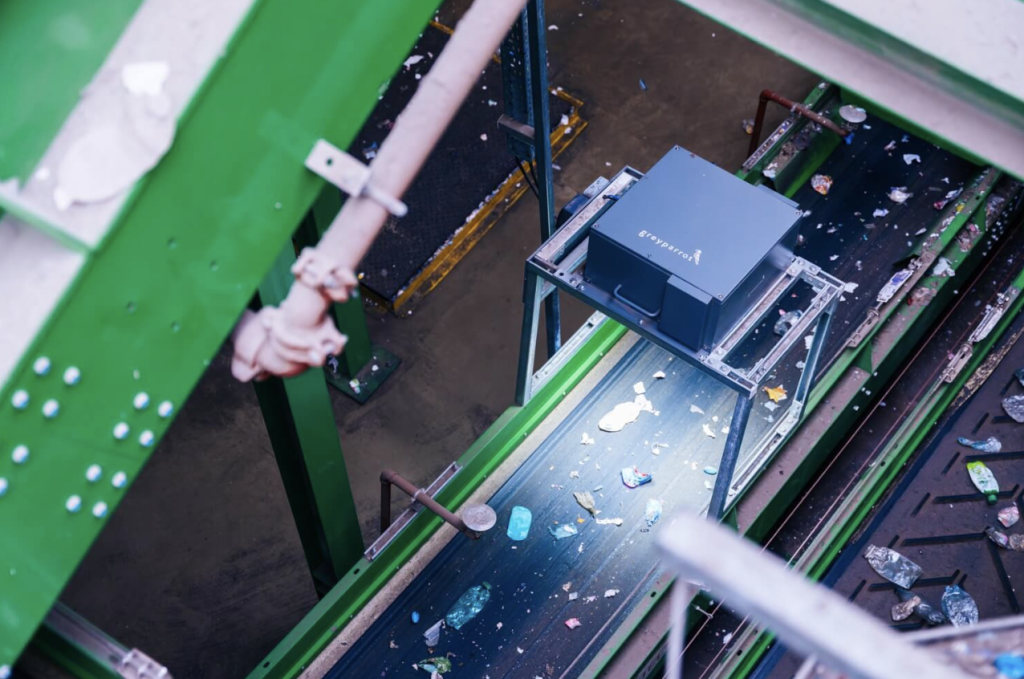
Greyparrot AI, an intelligent waste recycling company that was founded in 2019, “identifies 50 billion waste objects each year” through their AI-assisted analyzers across more than 14 countries.
The (Potential) Disaster
But there is a catch: AI itself has a massive carbon footprint. Hence, while some see it as a great opportunity for our fight against the climate crisis, there are also others that perceive it as an environmental disaster, and that it is not receiving the scrutiny that other energy-hungry technologies, like bitcoin, received. To say the least, their worries are justified.
Large Language Models (LLMs), like ChatGPT, require a significant amount of energy for training and operating (Luccioni, Viguier, et al., 2023). Compared to the less energy-intensive AI systems that are built only for doing specific tasks, these all-purpose LLMs are expected to have a steady increase in use (Luccioni, Jernite, et al., 2023).
Luis Cruz, a Computer Science professor in TU Delft who works for making AI more ‘Green’, says in an interview that “Training the ChatGPT 3.5 model costs around 500 tonnes of CO2 emission… roughly equivalent to 1000 cars each driving 1000 km”. This is a single ‘training’ session. It’s estimated that every 204 million inferences (processing queries by users) equals the total emissions for training a model (Luccioni, Jernite, et al., 2023), and together training and inferencing amount to nearly 90% of emissions of the model (Schroder Research, 2023).
It is already alarming, yet these models are expected to consume even more energy. You see, as better and better AI models were developed, this improvement has always been associated with increased complexity (Schwartz et al., 2020). For instance, while ChatGPT-3 had 176 billion parameters, its successor ChatGPT-4 has 1.76 trillion parameters now.
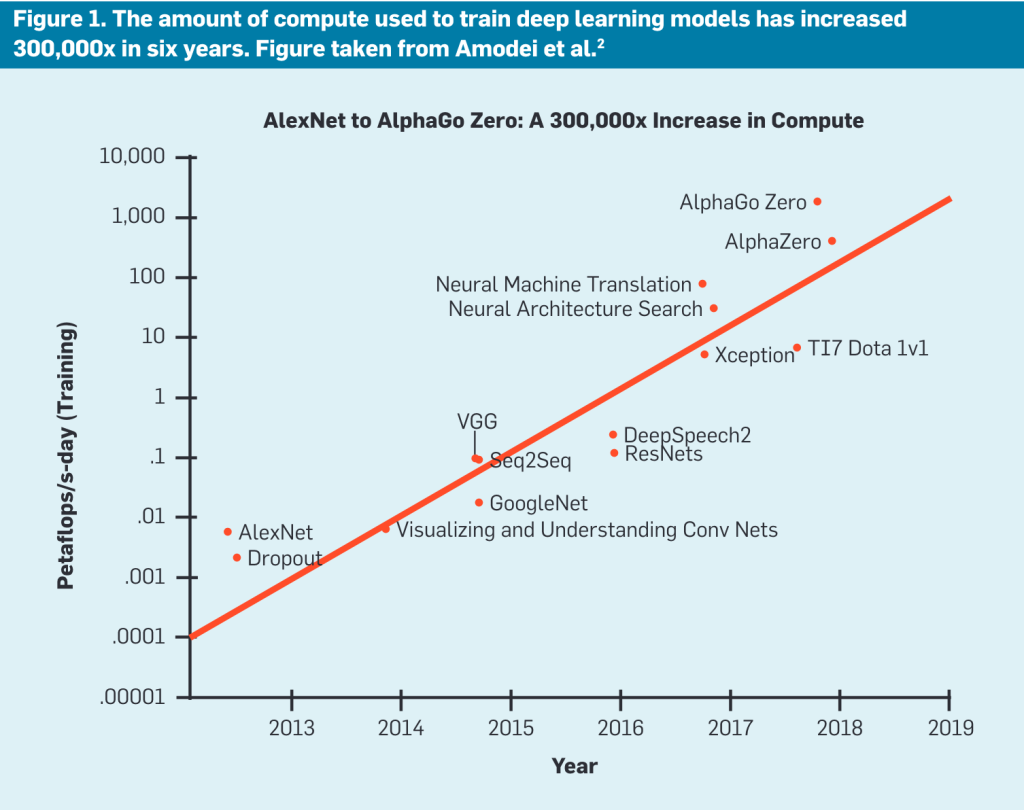
While complexity improves ‘model accuracy’, it is also associated with requiring more computations, and, therefore, with higher energy consumption. And many academics like Luis Cruz warn that this trend of building more and more energy-intensive models has to change.
In this regard, we believe in the potential of AI in helping us with the climate crisis, but we also believe that we need policy regulations to ensure that AI benefits the climate.
Green AI: Giving importance to efficiency
A research in 2020 found that there was an overall lack of attention to efficiency of AI models, in comparison to model accuracy (Schwartz et al., 2020). Increasing efficiency means having software and hardware that uses less computational resources as they normally would, which would lead to a lower energy consumption.
The researchers termed AI practices that take “efficiency as a primary evaluation criterion alongside accuracy” as Green AI, in comparison to, what they call, Red AI practices that solely attempt to improve model accuracy. Efficiency, and ‘Greenness’, in AI can be achieved on two levels: algorithmic and hardware.
By refining the computational efficiency of their algorithms, tech firms can lessen their energy footprint. Practices like model reusing and sharing, as well as improved data selection, utilizing high-quality data and discarding the unnecessary, can mitigate the need for energy-intensive operations (Schwartz et al., 2020). Furthermore, developing more specialized, instead of general-purpose models would also prevent unnecessary energy consumption.
On the other hand, advancements in energy-efficient designs and the integration of renewable energy sources are crucial. The competitive chip industry, with leaders like Nvidia and TSMC, is under pressure to innovate towards efficiency (Kantrowitz, 2023). Considering that GPT models draw significant energy in training and operation, using green energy for the data centers can also be a critical step. Strategic location choices for these data centers in green power grids, is essential and can further reduce AI’s carbon emissions.
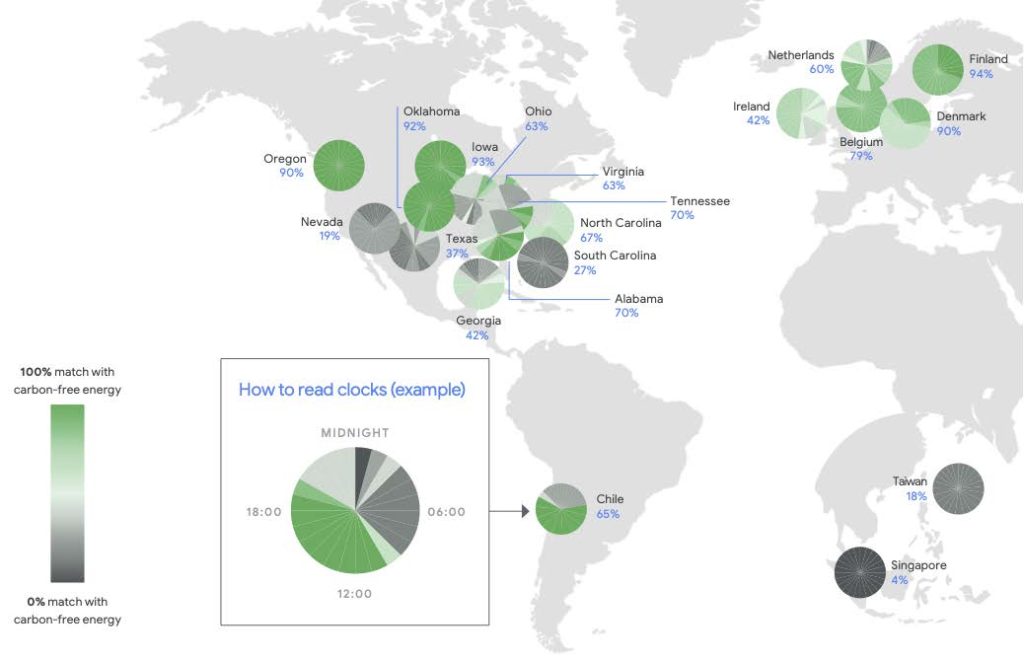
Unfortunately, there is currently a lack of an incentive structure for such Green AI practices that are necessary to make AI more climate-friendly. Companies achieve larger profits through models with better accuracy, and this pushes them to solely focus on this. We believe that this incentive structure needs to be changed by regulations.
Possible Regulations
Aside from CSR, governments and other institutions are uniquely positioned to create the boundaries for a healthy innovation climate. For hardware manufacturers there is a necessity to establish sustainable, intelligent, and cost-effective infrastructure. This could be achieved through innovative design that reduces energy consumption and carbon emissions. Policy can focus on stringent environmental assessment of the product manufacturing process, lifecycle, and recycling of materials (Strubell et al., 2019).
For tech firms, especially the gatekeepers, policies for energy requirements ensures software design is efficient. To enforce this perhaps the use of carbon accounting to measure and manage the emissions associated with AI operations can be utilized. Standardized reporting improves comparability and accountability, while the increased transparency will ensure public debate can be held (Cowls et al., 2023).
For academia funds and programs should be established that specifically aim to promote the development of research and metrics that focus on energy efficiency within AI. This could involve the creation of checklists for scientific publications, mandating the disclosure of important sustainability metrics, thus fostering a culture of transparency and accountability in research (Cowls et al., 2023). Such measures would encourage scholars to prioritize sustainability in their research endeavors, from the initial hypothesis through to publication. Establishing these standards will help discussion with tech firms on their responsibilities.
Would regulations be harmful for innovation?
Some might argue, especially the free market enthusiasts, that any regulation will hinder the development of this technology. One might say that the industry giants have the ability to take on the massive costs associated with the research and development of better AI models, and regulations would damage this effort.
Schwartz and colleagues also draw attention to another important aspect that Green AI would enable: inclusivity. Currently, the highly energy-intensive operation of the AI systems is also very costly as one might imagine. If you are a young researcher with brilliant ideas, you just need some teeny tiny sum of 100 million Dollars, which is apparently the training cost of ChatGPT according to Sam Altman.
In this regard, increasing the efficiency, and thereby reducing the costs, would allow more individuals to join in the development of these technologies. We do not believe that letting young researchers, enthusiastic entrepreneurs, and, overall, more bright minds to work on a technology would foster innovation, not hinder its development.
But wait, what if the current estimates are wrong?
In contrast with these views, some tech companies do not seem to hold a view that the energy consumption of AI will increase over time. For instance, a spokesperson for Google, Corina Standiford tells in an interview by The Verge that “The energy needed to power this technology is increasing at a much slower rate than many forecasts have predicted”. Or a recent publication by Google researchers state that “The carbon footprint of machine learning training will plateau, then shrink” (Patterson et al., 2022).
The big tech companies expect efficiency to be improved over time, essentially the technology solving the matter by itself. For instance, stating that “We have used tested practices to reduce the carbon footprint of workloads by large margins, helping reduce the energy of training a model by up to 100 times and emissions by up to 1,000 times”.
However, considering the ‘bigger is better’ mindset in the industry regarding the model sizes, without any accountability, there is no incentive for companies to turn their gaze from profit-bringing higher model accuracy to efficiency. It is thus not surprising to see that ICT (Information and Communications Technology) energy demand is poised to rise to 14% of global emissions in 2040 (Valerio, 2023).
Additionally, similarly, decreasing carbon footprint requires transparent reporting of emissions. Currently, both in the industry and in academia, there is a clear lack of such a practice. And we believe that regulations can definitely help with this.
An AI that benefits the climate
Overall, we believe that AI will benefit the climate change mitigation effort. That is for sure. However, we also believe that the current industry is focused on AI practices that are not aligned with this promise. We need ‘Green AI’, and this approach does not seem to be a compromise, but simply an improvement in terms of efficiency and inclusivity. In this regard, we need policy.
References
Cowls, J., Tsamados, A., Taddeo, M., & Floridi, L. (2023). The AI gambit: Leveraging artificial intelligence to combat climate change—opportunities, challenges, and recommendations. AI & SOCIETY, 38(1), 283–307. https://doi.org/10.1007/s00146-021-01294-x
Kantrowitz, A. (2023, December 15). The Competition is Coming for Nvidia. https://www.bigtechnology.com/p/the-competition-is-coming-for-nvidia
Luccioni, A. S., Jernite, Y., & Strubell, E. (2023). Power Hungry Processing: Watts Driving the Cost of AI Deployment? (arXiv:2311.16863). arXiv. https://doi.org/10.48550/arXiv.2311.16863
Luccioni, A. S., Viguier, S., & Ligozat, A.-L. (2023). Estimating the Carbon Footprint of BLOOM, a 176B Parameter Language Model. Journal of Machine Learning Research, 24(253), 1–15.
Patterson, D., Gonzalez, J., Hölzle, U., Le, Q. H., Liang, C., Munguia, L.-M., Rothchild, D., So, D., Texier, M., & Dean, J. (2022). The Carbon Footprint of Machine Learning Training Will Plateau, Then Shrink [Preprint]. https://doi.org/10.36227/techrxiv.19139645.v4
Schroder Research. (2023). AI revolution: What’s the environmental impact? https://www.schroders.com/en-gb/uk/intermediary/insights/ai-revolution-what-s-the-environmental-impact-/
Schwartz, R., Dodge, J., Smith, N. A., & Etzioni, O. (2020). Green AI. Communications of the ACM, 63(12), 54–63. https://doi.org/10.1145/3381831
Strubell, E., Ganesh, A., & McCallum, A. (2019). Energy and Policy Considerations for Deep Learning in NLP (arXiv:1906.02243). arXiv. https://doi.org/10.48550/arXiv.1906.02243
Valerio, P. (2023, April 24). Energy Efficiency Is Crucial for the ICT Infrastructure. EE Times. https://www.eetimes.com/energy-efficiency-is-crucial-for-the-ict-infrastructure/