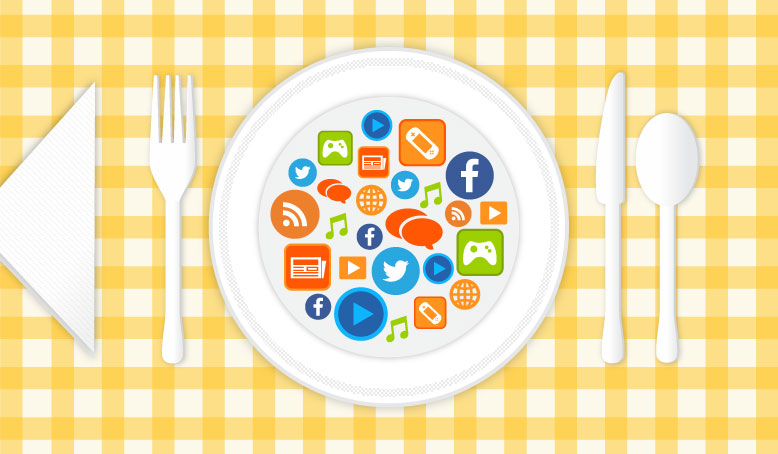
Have you ever heard the phrase “we are what we eat”? The truth is that this saying does not only apply to the physical world but also to our digital lives. Every day, we feed our brains with the content that we consume online, particularly on social media. Our reality is constantly being shaped by the AI recommendation algorithms that run these platforms, delicately trained, and optimized for maximizing user engagement and retention. This article delves into the hidden yet profound impact these AI systems have on societal discourse, where instead of broadening our horizons, they are funnelling us into more and more polarized bubbles.
The rise of algorithmic recommendations
AI recommendation systems on social media started as digital concierges, guiding users through the vast sea of online content. At their core, these algorithms analyse user behaviour – likes, shares, and viewing patterns – to tailor content that aligns with perceived preferences. The initial intent was straightforward: enhance user experience by delivering relevant, engaging content. However, as these systems evolved, their impact transcended mere content curation. Today, they play a crucial role in determining what news we see, the opinions we’re exposed to, and ultimately, how we perceive the world. This evolution has not only changed the way we consume information but has also raised critical questions about the societal implications of these algorithmic choices.
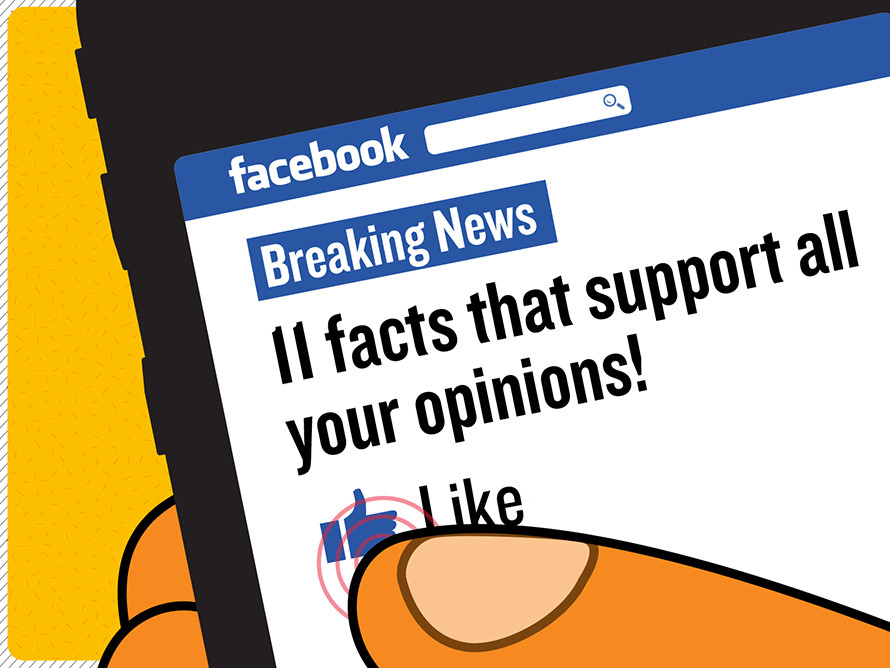
Image by Lamberto Ferrara
Echo chambers and filter bubbles
The heart of the issue lies in the underlying mechanism of these AI systems, their reliance on engagement metrics (like clicks, views, likes) to determine user preferences. This approach can be misleading, as high engagement does not always mean genuine interest or agreement. Frequency is not the same as preference. For example, sensationalist or clickbait content might receive frequent interaction not due to user preference, but due to curiosity or the provocative nature of the content. This misinterpretation leads to an overrepresentation of certain types of content, which might not genuinely align with the user’s interests. Because of their underlying mechanisms, a related problem arises. These algorithms tend to display more content that aligns with what users have previously engaged with, inadvertently reinforcing existing beliefs, particularly if the user interacts more with certain viewpoints or types of content. Over time, this creates a feedback loop, continually presenting the user with similar perspectives. The consequence is the formation of digital echo chambers, where exposure to a diversity of opinions is limited, and users find themselves in a cycle of repeated and similar content, reinforcing their pre-existing views. Studies, such as those conducted by Fabrizio Marozzo and Alessandro Bessi, have shown a marked increase in polarization attributed to social media use. These echo chambers do not just limit exposure to differing viewpoints but actively shape public opinion, often in more extreme directions. Similarly, filter bubbles are created as a result, where not only the social interaction is one-sided, but the feeds of these users will only show content conforming their believes. Moreover, these recommendation algorithms lack transparency and are often viewed as black boxes controlled by the Big Tech companies. Users rarely understand why certain content is presented to them, leading to a passive consumption of biased information. The absence of diverse perspectives is not just a theoretical concern. It has real-world implications, as seen in the spread of misinformation and the deepening of ideological polarization and societal divides. For instance, during political events, these systems can create a skewed representation of public sentiment, influencing voter perceptions and behaviour, and thereby challenging democratic practices.
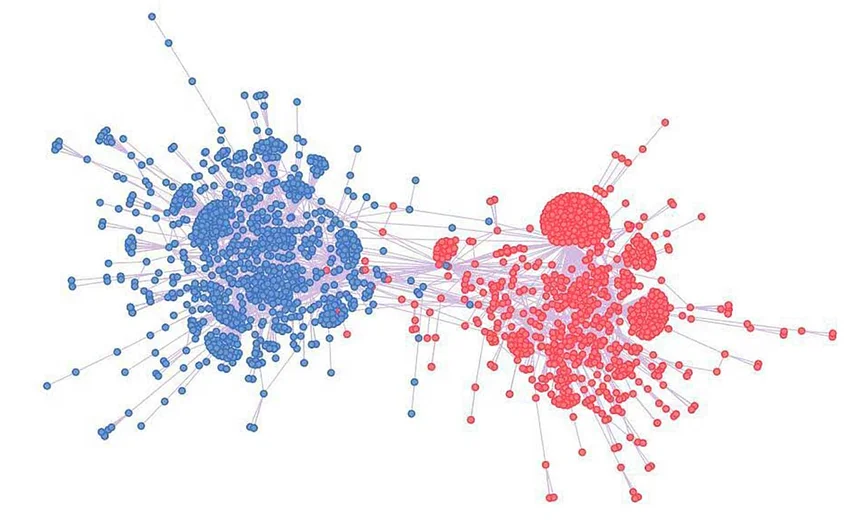
The dangerous aspect of these phenomena is its self-reinforcing nature. As users engage more with polarized content, the algorithms get better at serving more of the same, creating a loop that is hard to break. The danger is not just in the polarization itself, but in the erosion of a shared reality, essential for meaningful discourse and democratic processes.
So why use AI at all?
However, it is important to consider the other side of the argument. Proponents of AI recommendation systems argue that they significantly enhance user experience by efficiently filtering content in an overwhelmingly vast digital world. They also state that these algorithms help users discover content and communities that they might not have found otherwise, arguably enriching their online experience. This view overlooks the broader societal impact. Indeed, these algorithms can streamline our content search and connect us with like-minded individuals. However, this convenience comes at a cost, the cost of a narrowed perspective and an echo chamber that reinforces only existing beliefs.
Additionally, some experts contend that the algorithms themselves are not inherently biased; rather, they mirror the biases and preferences of their users. This argument that these systems are merely neutral mirrors reflecting user biases oversimplifies the issue. These algorithms are designed to prioritize engagement over accuracy or diversity. This design inherently favours content that is sensational or polarizing, as such content tends to elicit strong reactions and thus more engagement. Consequently, what starts as a reflection can quickly turn into amplification, where extreme views receive disproportionate visibility.
There is also an argument to be made about personal responsibility in media consumption. Users, as active participants, have the power to diversify their digital diet, stepping out of algorithm-curated comfort zones. For us, this call for personal responsibility in media consumption, while valid, ignores the subtle and often subliminal influence these algorithms have. The average user, unaware of the intricacies of these systems, may not realize the extent to which their views are being shaped. This lack of transparency and user awareness makes it challenging for individuals to break free from the algorithmic bubble. Thus, while AI recommendation systems have their benefits, their role in promoting polarization and limiting diverse viewpoints cannot be understated. The need for reform in these systems is imperative, aiming for a balance that fosters both personalization and exposure to a range of perspectives.
Conclusion
The debate around AI recommendation systems in social media is not just black and white. While they offer undeniable benefits in content curation, the consequences of their unchecked influence on public discourse are too significant to ignore. As we navigate this digital era, it’s crucial to strike a balance between algorithmic assistance and conscious media consumption. This article serves as a call to action for both social media platforms to enhance transparency and algorithmic diversity, and for users to actively seek varied sources of information. Only through collective efforts can we ensure that our digital future is not one of divided realities, but a tapestry of diverse, yet harmonious, perspectives. It is crucial to steer away from the current trajectory, leading us to more mindless and curated consumption of content, and start making our digital diet as varied and balanced as our physical one, for the sake of a healthy, well-rounded societal discourse.
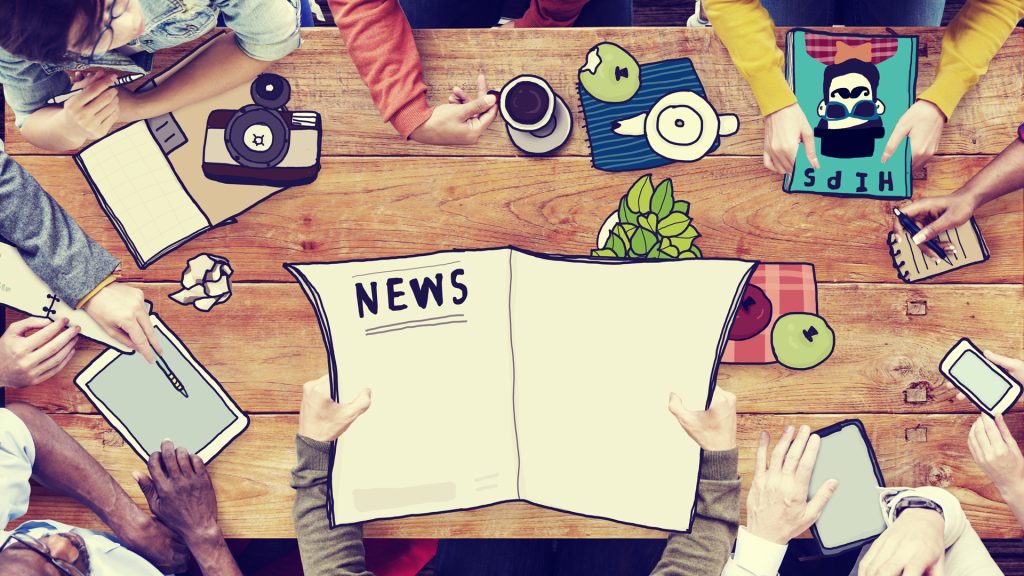