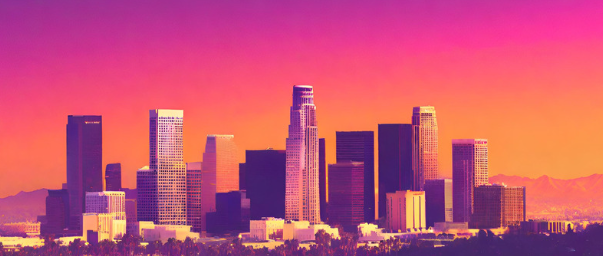
Imagine living in a city where law enforcement is pioneering predictive policing to fight crime, with the Los Angeles Police Department (LAPD) leading the way. In the heart of this metropolis, the LAPD has deployed avant-garde programs such as PredPol and Operation Laser, which boast of their ability to predict future crimes using historical data and software. The city wants to predict where crime will happen. Have you ever been in contact with law enforcement? Or has your family? Then you might already have some points added to your name. Score enough points and you’ll end up in the Chronic Offender Bulletin (COB). The COB is a list of individuals who are deemed to be at a high risk of reoffending and therefore require close monitoring.
As the concerned people of Los Angeles lived this scenario, they advocated for more transparency while pulling back the layers of these programs. They unveiled documents that shed light on the troubling consequences of predictive policing, particularly in the over-policing of black and brown communities. Creating an even more biased and racism perpetuating system.
Although designed to be precise and innovative, the LAPD’s flagship programs instead reaffirmed existing biases and policing patterns. Operation Laser, launched in 2011 with the intention of carefully singling out ‘criminals’, relied on data that could be considered malicious, creating a self-perpetuating cycle of crime reports and arrests in targeted areas. Similarly, PredPol’s application of an earthquake prediction model to crime failed, resulting in flawed predictions and a lack of tangible benefits, prompting several police departments to terminate their contracts with the company.
The LAPD announced that they too had terminated their partnership with Predpol and Operation Laser as they faced increasing criticism and empty promises of improvement. However, this has brought about a change rather than the conclusion of the tale. Following it, a brand-new initiative called Data-Informed Community-Focused Policing (DICFP) was introduced, emphasizing community cooperation and transparency. Experts, however, contend that the new campaign is strikingly similar to its predecessors, leading to worries that it could be merely a rebranding attempt rather than a significant shift.
These cases sketch some problems that extend through predictive policing, from biased targeting and lack of precision to the persistence of questionable practices. This essay examines predictive policing and its historical bias. We argue for the need to move away from historical bias and propose the use of new, less unbiased data in the creation of predictive AI models for policing.
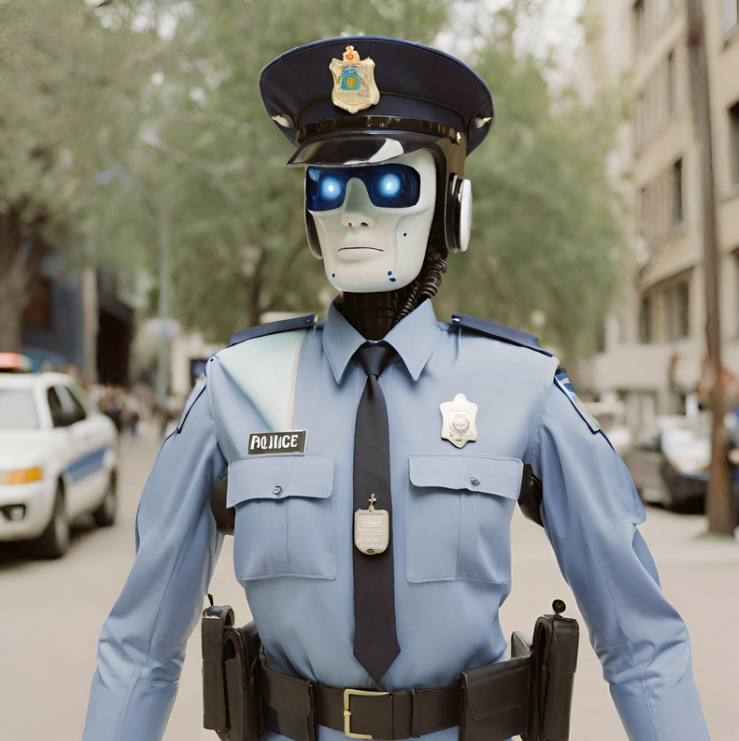
The Role of Bias
All current predictive policing systems are built upon data from the past 50 years, a period marked by significant inequalities and privileges. Research, including those published in Nature, acknowledges the inevitable bias inherent in humans, a trait we cannot simply shed. Recognizing this, we can strive for solutions that look beyond this bias. However, the question arises: how does this human bias reflect in predictive policing systems which are often deemed objective? It’s a common misconception that machines, lacking the ability to have opinions, are inherently unbiased. Yet, predictive policing systems can and do exhibit bias, sometimes confirming or even amplifying our own human prejudices, as highlighted in studies by Richardson et al. (2019).
When a police department has a history or convictions of unlawfully biased arrests, the data from such arrests is termed “Dirty Data.” Currently, there are no laws or rules that prohibit the use of this “Dirty Data” in training predictive models for policing. This results in models that perpetuate and predict in line with the biases inherent in the data they are fed. It is a simple “Bias in, Bias Out” phenomenon, and because there are no checks nowadays to any of the data that gets in, the bias lives on.
The Usage of New Data
To achieve a fair and unbiased predictive policing system, it is crucial to prevent biased, unchecked, human-generated data from being included in the training process. Instead of relying on historical crime data, a more forward-looking strategy should be adopted, using new data from the present. This approach will create a clean slate for AI models and minimize the impact of historical bias. People are never completely unbiased. However, we believe that today, people are more aware of the biases that exist in our world than they were 50 years ago. This awareness is already a step towards making fairer judgments.
We advocate for the use of new data in predictive policing systems to eliminate the biases inherent in historical judicial practices. Our understanding of policing has evolved dramatically over the past 50 years, recognizing many previous practices as deeply biased. Continuing to use this outdated data undermines current efforts to implement fair and effective policing.
Modern data collection is not only more comprehensive but also reflective of today’s societal and legal standards. As laws and ethics evolve, so too must the data that informs our policing strategies. By leveraging recent data, we align policing with contemporary values, ensuring a fairer and more accurate reflection of our society. In essence, using new data in predictive policing is not just a technical necessity but a moral imperative, vital for a justice system that evolves alongside our societal progress.
While the shift to using new data in predictive policing systems is advantageous, it’s not without its challenges. One primary concern is the time required to develop reliable models with this fresh data. This slower pace, however, should be viewed not as a drawback but as a necessary diligence in pursuit of fairness and justice. When decisions have life-altering implications, as they often do in the courtroom, a cautious and measured approach is crucial. The slower evolution of these models reflects a commitment to accuracy and ethical responsibility, underscoring why new data is preferable.
However, it’s crucial to acknowledge that even recent data from police departments may not always represent fairness. Recent reports from entities like the UN highlight ongoing discrimination issues in various U.S. regions, suggesting only incremental progress towards equity. This reality raises a question: why rely on current data if it’s potentially as flawed as historical data? The answer lies in the methodologies of data collection and processing. In addition to being gathered more thoroughly, modern data is also carefully processed and filtered.
It is true that we are still far from having a fully equitable judicial system, and we should proceed cautiously and sensibly when interpreting the data that is available. But it’s also true that this journey is marked by progress. By putting our faith in this progress and still maintaining strict guidelines for data processing, we guarantee that newly developed predictive policing systems are based on the most recent, thoroughly examined data. This strategy strikes the right balance between the demand for progress and the requirement for careful and sensible data use.
The Responsibility Lies With All of Us
As predictive policing was brought to life thanks to AI models, it is important to be aware of the responsibility that comes with designing AI models. Altogether, we and many others should keep highlighting the issues that arise with implementing AI in policing. By doing so, this hopefully forces reflection of all parties involved, which ultimately will be everyone, as it integrates in daily life more and more. The proposed idea of using new, less biased data in predictive AI models for policing, emphasizes the responsibility and influence we have as AI specialists. All together we are navigating a fast-developing world where we need to keep questioning what kind of AI future we want to create and how we can contribute to steer it towards an ethical and fair one.
In conclusion, our examination of predictive policing reveals that new, more recent data must be incorporated into AI models in order to overcome the urgent need to abandon historical bias. This transition is essential not only for upholding fairness and accuracy in law enforcement but also for reflecting our evolving societal and legal standards. While challenges exist in adopting new data, including the time required to develop reliable models and the ongoing struggle against discrimination, these hurdles do not outweigh the benefits. A careful and considered approach to data processing and model development can lead us towards a more equitable and just policing system. The journey towards an unbiased, effective predictive policing system is ongoing, but with a commitment to using less biased, current data whilst keeping a close watch on the rapidly changing field of artificial intelligence, we can steer towards a future where justice is not just an ideal but a reality. The responsibility lies with us as AI specialists, policymakers, and community members to shape a future where AI in policing upholds the principles of fairness and equality for all.