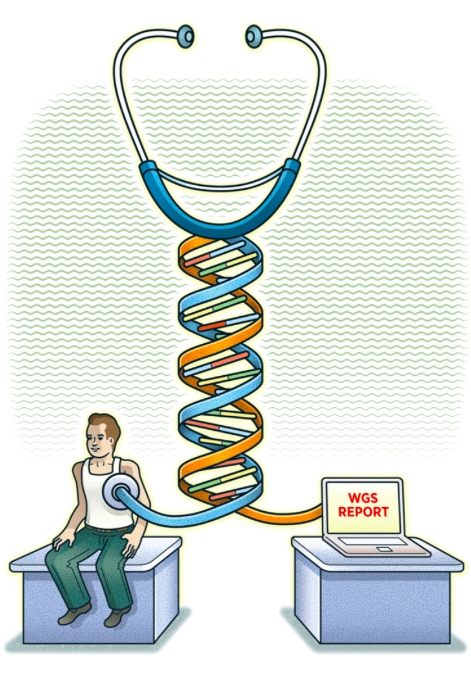
Source: Market Stats News
Personalized experiences arise everywhere around us. Our choices of TV shows to stream, music to listen to, and purchases to make are all influenced by recommendations based on our personal data and of people around us. Smart watches track our health statistics, such as our heart rate, the amount of hours we sleep and the calories we burn. As consumers, we use this information to optimize our daily lives. We adjust our workouts by checking our heart rate, we take power naps if we did not spend enough time in REM sleep last night and we pick a meal that fits our calorie balance of today. But there is more than just personal profit to be gained. Combining personal data of people is extremely valuable, as analyzing patterns in large datasets could give insights that would not be gained otherwise. This is widely used as a marketing strategy that adopts decision making based on data analytics rather than human intuition or experience. Apparently Aristotle knew all along: the whole is greater than the sum of its parts.
And it is not just for marketing purposes that the collection and analysis of personal data is meaningful. In the medical industry, it contributes to a profound shift in medicine, known as personalized medicine. This transformation is built upon the idea of improving our healthcare system by individualization. Personalized medicine substitutes current methods based on traditional statistics to diagnose. It provides alternative methods that take more detailed information of individuals into account, such as the variety of genes, environment and lifestyle. The collection of these types of detailed information is known as big data: large datasets that cannot be analyzed, searched, interpreted, or stored with traditional data-processing methods. Recent attempts to implement personalized medicine and to use personal data to optimize health care have proven to be rather expensive and inefficient. Medics face an overwhelming amount of patient’s data and an excessive time pressure for data entry, while they have no access to effective analytical tools to support them. This is where Artificial Intelligence (AI) offers a solution. AI analytics are used to analyze these large collections of personal data and is the key technology that enables this shift towards prevention, personalization and precision.
“The whole is greater than the sum of its parts.”
Aristotle, philosopher
AI analytics
It is mainly Machine Learning (ML), a form of AI that contributes to the developments of these intelligent systems towards personalized medicine. In ML techniques, a computer is fed data, say, images of animals with their corresponding names as labels. Based on this knowledge, the computer program ‘trains’ itself to recognize what would look like these animals. Once the algorithm is trained, it can classify objects. When it is fed an unknown image of a bird, for example, it will assign a label to this image based on the known images of animals that have the highest similarity with the new image. If the algorithm assigns the label ‘bird’ to it, the task is accomplished. With the right software and a lot of structured data for the computer to train itself on, the program can tune itself to identify animals in images. So, it can explain some relationship between variables.
In a medical context, ML techniques are used to identify trends, patterns and anomalies in the data that can help experts make better-informed and more accurate decisions and predictions about some treatment, rather than simple estimated score systems. Furthermore, such systems can find new patterns in the data, and therefore supports an acceleration of new drug research. Since AI is developing very fast, a future of effective and personalized healthcare is achievable. And it is not only achievable, it is also desirable.
Targeted treatments
Nowadays, research in medicine uses population-wide models that are applied to the population as a whole. Clinical trials aim to evaluate the effectiveness of treatments or drugs based on how the majority of a group responds to them. Furthermore, traditional statistics are used to predict how the average number of people of that group will respond to a certain medicine. In this approach, individual differences in treatment responses are ignored while it is widely acknowledged that the effectiveness of a certain treatment can differ among individuals. Patients could be denied certain treatments because care plans are based on averages of populations, making it more difficult for an individual to get healthcare that is tailored to this individual. For that reason, Donna Dickenson, professor of Medical Ethics at the University of London describes traditional medicine as “one-size-fits-all medicine”.
Source: TEDx
Personalized medicine, on the other hand, is radically different in nature by focussing on patient-specific treatments that will give the best possible outcome based on personal information. Recent large-scale genomic sequencing analyses have revealed enormous genetic diversity between individuals. For example, in diseases that are driven by genetic events such as cancer, mutations that drive individual tumors can already be unraveled by genomic sequencing analyses. There is an increasing amount of genetic data available on which a more targeted choice of treatment can be based. However, it has become challenging for physicians to analyze the large amounts of data that is necessary to accomplish this.
And at this point AI proves its indispensability. AI data analysis techniques have the ability to collect, store, normalize and trace data in ways that are beyond human capabilities. This way, decisions on the type of care for patients can be optimized. Recent studies have already shown the value of AI driven personalized medicine. For example, a 2021 study shows that using ML to select patients suffering from COVID-19 in the evaluation of the medicine can improve a targeted prescription of these medicines. Two medicines, Remdisivir and Corticosteroid, were both shown to be effective treatments for people with COVID-19 symptoms for a group of patients, while they did not prevent other groups of patients from progressing to a severe stage in the disease. By evaluating the performance of Corticosteroid and Remdisivir based on a patient’s survival time, traditional methods were used and no significant increased survival time was found for the use of either of these medicines. However, using an ML algorithm to select patients to evaluate the performance of the medicines, there was a significant increase of survival time found for both medicines. The selection of the groups was based on more personal data and led to a more specific group of people to evaluate the performance of the medicine. Therefore, analyzing datasets containing personal data allows a more targeted use of medicines, using a patient’s information to choose medicines that are most likely to be effective. On top of that, since AI algorithms can improve the understanding of human health by identifying patterns in data, features of a particular disease course can be identified.
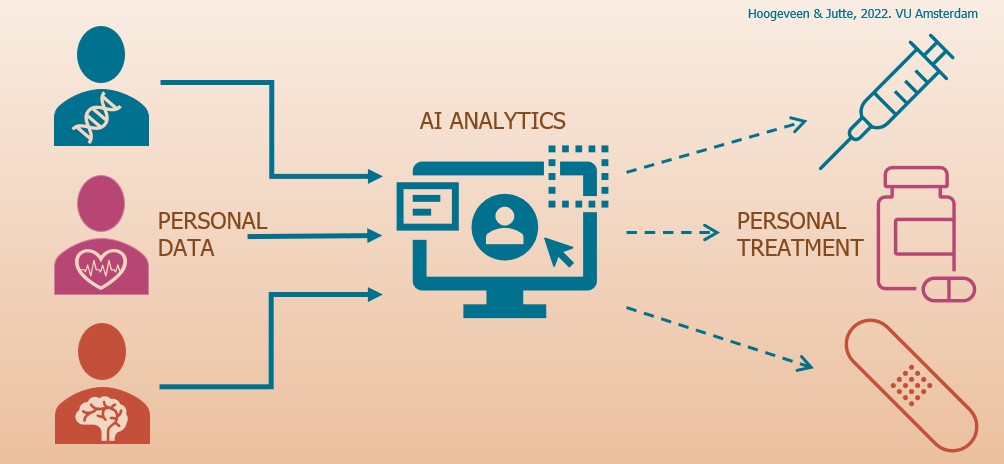
Focus on disease prevention and monitoring
Applying AI techniques in personalized medicine will not only optimize treatment procedures. It will also cause a shift of focus to disease prevention instead of treatment because of the emerging opportunities to recognize disease traits in early stages. By recognizing gene mutations that are known to potentially cause illnesses, AI analytics will enable the prediction of health conditions before they arise. This way, it allows clinicians to take measures to increase the chance preventing diseases. For example, big data analytics have the potential to identify unknown risk factors of cardiovascular diseases.
On top of that, the identification of patterns in patient’s data supports the ability to monitor the development of a disease. The more information on the development of a disease, the more chances there are to take preventative measures or even to introduce treatments before a patient shows symptoms. Imagine different patients suffering from cancer. These patients may experience unique genetic changes in their cancer cells or different responses to the cancer due to genetic differences. Both factors might affect how their cancer progresses and by using this information, the diseases can be monitored and lead to personal adjustments of the treatment. This way, the chance of getting a certain disease might be decreased or controlled in early stages.
Reducing healthcare costs
The current population based model is a practical method to deliver care to every individual member within the selected community. Delivering care this way is cost-effective and it is quick to respond to immediate healthcare. However, it fails to take into account individual needs. By making medical decisions and interventions based on the prediction of individual disease responses, personalized medicine safeguards and builds upon personal characteristics. This way, AI has the potential to make our healthcare system more efficient and less expensive by potentially reducing medical errors that can lead to deaths and unnecessary costs. It has the possibility to lower healthcare costs by optimizing and effectively developing therapies and by taking over tasks from medics. On top of that, other sectors associated with healthcare could benefit financially from implementing AI in patient care. It could for example support insurance companies to predict risks of individuals and population groups. Finally, AI analytics can be used to run various experiments to improve the efficiency of clinical trials. Implementing a smarter design of clinical trials by selecting groups based on likelihood to respond well, time and costs needed to execute these trials can be reduced.
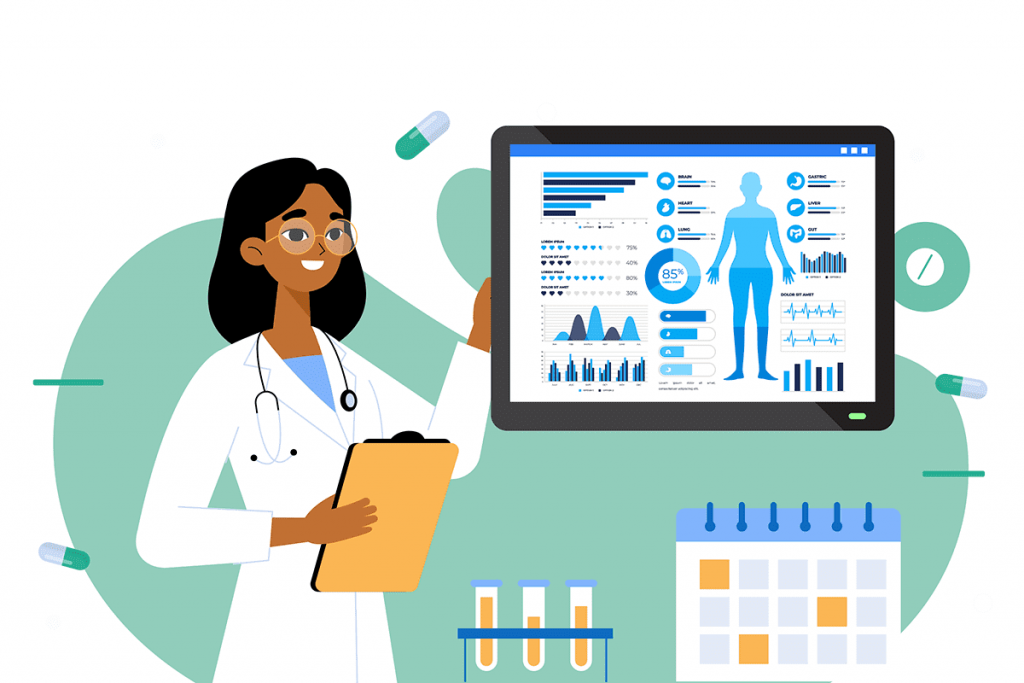
Source: Springboard
Privacy issues
Personalized medicine raises some concerns too. To optimize individual care, access to big data is required to perform useful analyses and identify patterns of personal characteristics and disease developments. It does not come as a surprise that privacy issues arise from this need for personal information of individuals. Health tracking applications collecting health data are known to share data with third parties, often without the users consent. It is a valid concern that supplying personal data might violate the privacy of individuals.
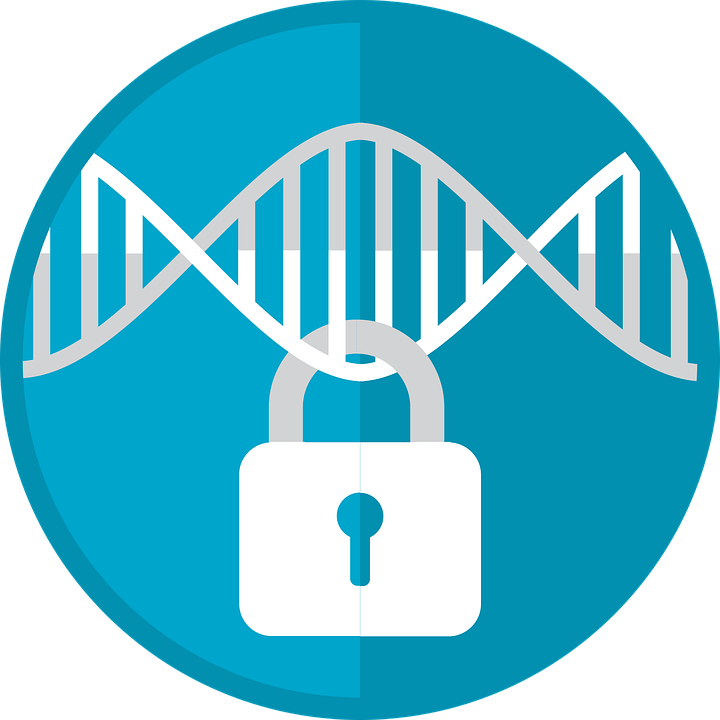
Source: The Medical Republic
However, it is possible to create circumstances in which these concerns can be eliminated. These circumstances are characterized by targeted legislations that can ensure patient confidence in digitalized healthcare. Research shows for example, that in order to share their data, rare disease patients want specific requirements in order to respect their privacy regarding the use of their data. Therefore, for the implementation of well organized AI systems to optimize healthcare, a cautious approach that reduces the risks of privacy violation is necessary. For example, data banks should be protected and algorithms applied to personal data should be open source and explainable. Many efforts have been made to make sure that legislation around health information privacy matches the current situation, such as the California Consumer Privacy Act and the European General Data Protection Regulation. On top of that, AI technologies can even preserve privacy.
Increased bias
A second, widely acknowledged problem of implementing personalized medicine is the possibility of biased AI systems. The possibility of algorithms increasing existing biases is widely acknowledged as a problem that occurs in all applications of AI. AI applications in digital health systems have been criticized for propagating existing biases. The problem lies within the enhancement of biases in the training dataset of an algorithm. AI can increase those biases because it learns the patterns from this data and makes predictions based on these patterns. For example, black women with breast cancer have shown to be less likely to be tested for high-risk mutations than with white women. While both groups of women carry a similar risk of such mutations, the risk of breast cancer for black women is more likely to be misinterpreted based on algorithmic genetic tests.
Although the possibility of biased AI raises valid concerns, biased data in the medical field has always been a problem. It is known that data used in healthcare can be biased and concerns about potential biased AI is similar to concerns raised in clinical trials nowadays. Most medical research is done on a non representative group leading to wrong diagnoses for underrepresented groups, such as women. On top of that, biased medics could negatively evaluate a person on the basis of irrelevant characteristics such as race or gender.
Therefore, fighting existing biases in data sets that are used in medicine remains a challenge. By strategically deploying AI and selecting data sets in a more secure way, biases can be mitigated. Moreover, AI can help to identify biases in healthcare and that may help identify interventions that could help correct biased clinician decision making and possibly reduce health disparities. AI decision support tools could be used to identify real-time bias in clinicians’s decision makings. Many non-medical factors affect the decision making, which can also lead to bias. If rational AI predictions and clinicians’s decision makings differ in these situations, clinicians could be alerted immediately about their potentially biased decisions. Other possible solutions could be to address bias in healthcare by using sensitivity checks, including simulated data sets to determine how robust predictions are to omit bias. Furthermore, by applying ML algorithms to find potential biases, also known as AI audit, the biases can be reduced by detecting it and deleting it.
Regulation, education and implementation
It is clear that realizing personalized medicine has many advantages for everyone involved in healthcare. It enables targeted treatments based on individual patient characteristics, rather than on population averages. With data analytics, the focus can shift from disease treatment to preventing and monitoring diseases in early stages. It could prevent people from suffering by taking precautionary measurements and if treatment is needed, it can be adapted to personal needs more easily. This will support accelerated and specific clinical trials and specialized care, which in turn leads to a decrease of healthcare costs. Challenges faced are privacy issues and deeply rooted biases in the medical field. These problems are to be taken into account and tackled as much as possible. By strategically deploying AI and carefully selecting underlying data, algorithm developers can mitigate AI biases. Along with improving abilities to address biases, AI could reach its fullest potential by helping to improve diagnosis and prediction while protecting patients. Regulations enforced by new or extended laws will make sure that privacy requirements are met. The increasing attention for and developments of legislation around these issues are promising for the near future.
However, regulation is only part of the solution. Gaining knowledge about personalized medicine and the use of data and analytics is just as important for everyone involved. This can be realized through education about the different aspects of personalized medicine for those involved, from doctors to patients and from data analysts to policymakers. As Ashish Jha claims, the potential of AI is equally big as its challenges. This way, AI could assist medics in making clinical decisions. To be able to deliberately transition to personalized medicine, it is therefore necessary to understand both the strengths of AI and its weaknesses.
“The potential of AI and the challenges of AI are equally big”
Ashish Jha, director of the Harvard Global Health Institute
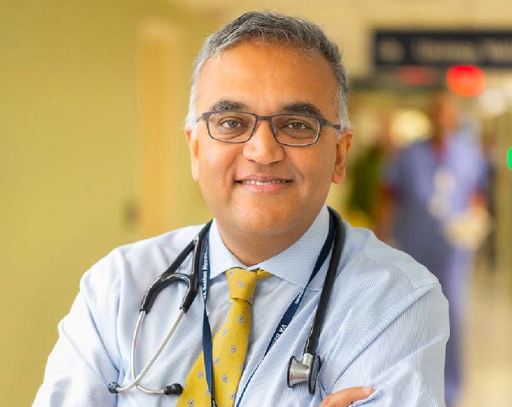
Source: The Harvard Gazette
Eventually, the actual implementation of personalized medicine and the associated AI systems is what is left once appropriate legislation is established and those involved have sufficient knowledge to achieve goal-oriented and ethical personalized medicine. There is still a lack of validated impact of AI on clinical practices in healthcare, that can partially be attributed to the incompetence of doctors to correctly interpret data analyses. It is important that a shift will take place, so that AI can be beneficial for healthcare in the way it is shown how AI can be beneficial for healthcare with personalized medicine. It is time to regulate, educate and implement. As large biomedical datasets are available, we lag behind events and there is an urgent need to deliberately integrate AI into healthcare. Medical opportunities created by technical developments in the field of AI call for clear legal boundaries and human efforts to get a grip on the technologies available. This way, personalized medicine will be the remedy for healthcare that will benefit society as a whole.