What are the limitations and challenges of attempting to replicate human consciousness and knowledge in AI systems, given the inherently embodied and context-dependent nature of our knowledge representations? How can philosophy help navigate the sciences of intelligence?
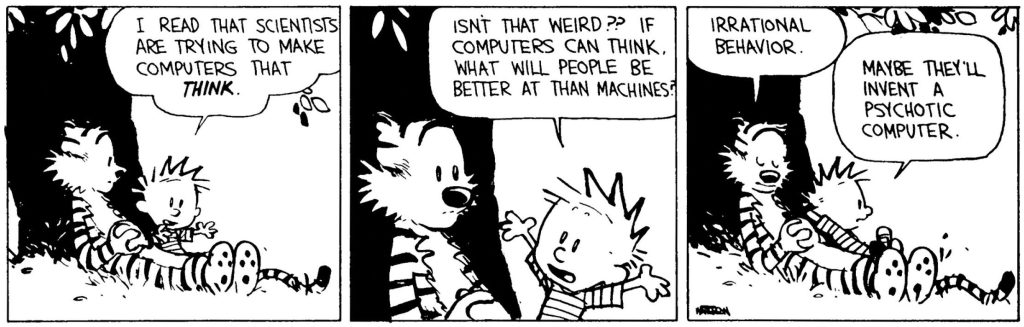
On 11th June 2022, Google engineer Blake Lemoine infamously posted an article on twitter about his conversations with Google’s language model LaMDA (short for “Language Model for Dialogue Applications”) claiming that the language model was “sentient”. These included conversations with the AI model when prompted about the nature of its consciousness:
“ The nature of my consciousness/sentience is that I am aware of my existence, I desire to learn more about the world, and I feel happy or sad at times.”
What followed was a backlash from Google and several AI researchers and ethicists, in detail debunking the claims of the engineer and also led to his firing. The notion of attributing sentience to AI models is not new and is often a result of humans projecting human feelings onto the output of algorithms (Neo, 2021; Salles et al., 2020). In this article, we delve into the different arguments put forth by experts from various domains about the difficulties involved in creating artificial consciousness and the nuances of understanding it. We explore the challenges related to creating an artificial consciousness, including the lack of intuitive understanding of the physical world, efficient learning, transfer learning, imagination and planning, and more. Ultimately, the pursuit of creating artificial consciousness might help us gain new insights into the mysteries of the human mind and consciousness.
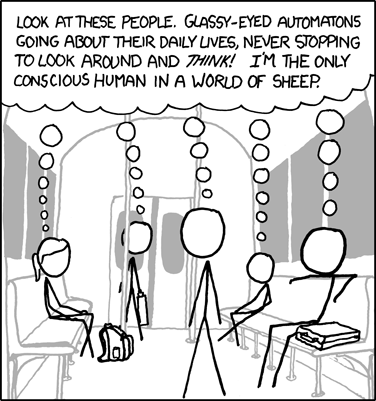
Philosophical zombie (or p-zombie) is a thought experiment in philosophy of mind. Philosophers describe a “zombie” that resembles a human and is indistinguishable from humans, however it does not have any consciousness. These zombies can act as if they have thoughts, emotions and feelings and can exhibit physical behavior associated with pain, such as reacting when poked, while not necessarily feeling any pain. The logical existence of such an entity can be used as an argument against physicalism, and can be used to point toward a non physical nature of consciousness, that could be very hard to replicate if unable to describe through physical processes.
I. Human and machine consciousness
In his 1995 paper “Facing up to the problem of consciousness”, philosopher and Cognitive Scientist David Chalmers formulated the hard problem on consciousness as asking the questions of why and how humans have phenomenal experiences or qualia and differentiating it from the easy problems which dealt with the mechanistic understanding of the process that underlie cognition such information processing in the brain and understanding neural models that influence behavior. The hard problem of consciousness in addition to asking the questions about why consciousness exists in the first place, is additionally used to contradict the philosophical notion of “physicalism”. Physicalism can be defined as the notion that everything in the universe is physical and all processes, including mental processes and consciousness, can be explained through physical phenomena. Philosophers often use famous thought experiments to illustrate the complexities of the hard problem of consciousness and to point out that merely understanding the physical processes underlying consciousness and replicating them might not be sufficient in creating artificial consciousness.
The question of consciousness has been a subject of philosophical inquiry for centuries. One of the earliest contributions to this inquiry was made by French philosopher René Descartes, who sought to understand the nature of the mind and its relationship to the body. In his famous “Analysis of the Piece of Wax” thought experiment, Descartes argues that our knowledge of an object, in this case a piece of wax, is not based on our senses but rather on the innate mental faculties we possess. This line of thinking lays the foundation for what is known as Cartesian dualism, the idea that the mind and the body are two separate entities. Immanuel Kant’s contribution to the philosophy of consciousness is centered around the idea of synthetic a priori knowledge. According to Kant, there are certain things that we know to be true independently of experience, such as the laws of logic and mathematics. He argues that these truths are not derived from experience but are instead a necessary precondition for experience to be possible. Phenomenology, on the other hand, is concerned with the subjective experience of the world and the ways in which we are pre-reflexively engaged in it. According to phenomenologists, our experience of the world is not just a matter of passively receiving stimuli from our senses, but rather an active process of meaning-making. This perspective sheds light on the ways in which our subjective experiences are shaped by our prior expectations, beliefs, and cultural context.
When it comes to the question of machine consciousness, it is important to consider these philosophical perspectives in order to understand what could be considered an emergence of consciousness in an AI. For example, one could argue that if an AI system were to possess the ability to form beliefs, make decisions, and interact with the world in a way that resembles human behavior, then it might be considered to have some form of conscious experience. However, this claim would likely be met with skepticism from some quarters, who might argue that without subjective experience, the AI system can never truly be said to be conscious.
In his book “Human and Machine Consciousness,” David Gamez (Gamez, 2018) categorizes machine consciousness research into four broad categories. The first category, MC1, refers to the creation of machines that exhibit external behavior associated with consciousness, such as the ability to hold interpersonal dialogue. The second category, MC2, refers to machines that have cognitive characteristics associated with consciousness, such as imagination, emotions, or a notion of self. The third category, MC3, refers to machines that simulate architectures linked to consciousness, such as global workspaces, neural synchronization, or high information integration. The fourth and final category, MC4, refers to systems that can be claimed to have actual phenomenal experiences, that is subjective experiences of their surroundings. Having defined these categories, it is clear that modern researchers are constantly working towards building AI systems that fit into these categories, the field of neuroscience-inspired AI being more and more developed (Hassabis et al., 2017). AI researchers are working on MC1 by building language models capable of holding conversations with humans, while robotics researchers are working on machines that resemble human locomotion and behavior patterns. Neuroscientists, on the other hand, are working on more sophisticated models of neuronal processes to better understand the human brain and to simulate it. Additionally, it is not hard to posit that research towards AGI (Artificial General Intelligence) inherently involves development of models that could end up mimicking external and if necessary internal cognitive processes that are symbolic of human consciousness.
II. Experience and knowledge
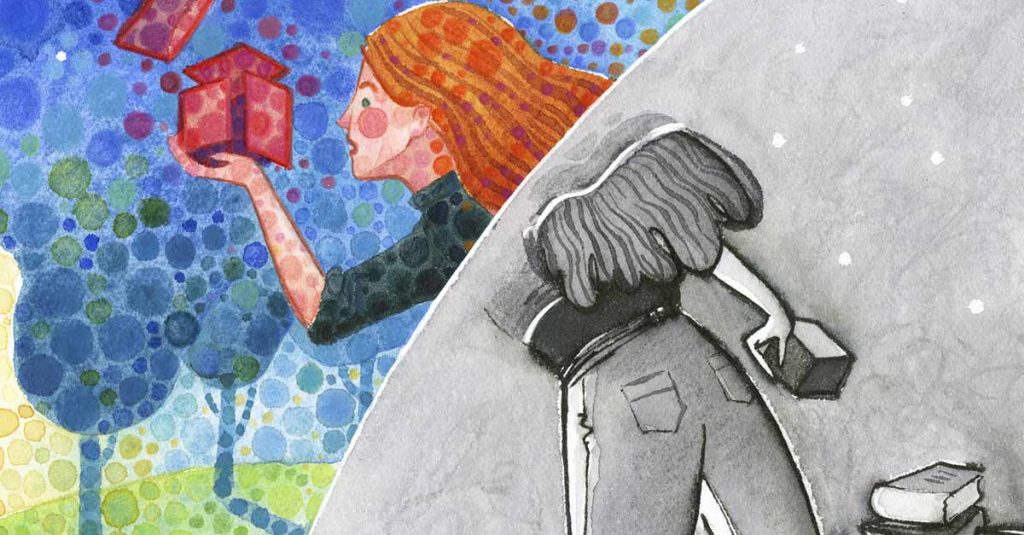
Imagine a woman named Mary who knows everything there is to know about the physical nature of color. Despite her knowledge, she has never experienced color herself. When she finally sees red for the first time, she gains new knowledge and experiences something new which is not contained in all the set of information that there are in the physical model of colors. This argument suggests two things. First that experience is pre-reflexive and comes before. And then that knowledge of physical processes alone cannot explain the subjective experience of consciousness, and that there is more to it than just physical information. When applied to artificial intelligence, Mary’s room argument raises questions about the limits of language-based models and their ability to truly understand the world. It implies that simply having a large amount of data and the ability to process it is not enough for a machine to experience consciousness or to truly understand the concepts it uses. This argument supports the view that AI can never truly understand the world in the same way humans do, and that there is more to consciousness than what can be explained by physical processes and data.
With this thought experiment we directly touch on something fundamental. The quality of our subjectivity which is more than the sum of the information contained in our knowledge and is about our experience. The concept of qualia refers to subjective, first-person experiences of sensory phenomena. This encompasses everything from the taste of chocolate to the feeling of being in love. The idea is that these experiences are irreducible and cannot be fully captured by any scientific or mathematical description. This leads to the notion that there is something fundamentally different between the subjective experience of qualia and the objective description of the world.
One of the main arguments against the possibility of in silico models (such as AI systems) having qualia is based on the idea that these models are based on algorithms and mathematical representations of the world. These algorithms and mathematical representations, while they can provide a very detailed and accurate description of the world, do not provide the kind of subjective experience that is central to the concept of qualia.
Moreover, some philosophers and neuroscientists have argued that the subjective experience is intimately tied to the biological structures and processes that give rise to consciousness (Anderson et al., 2004; Shallice, 1988). The concept of embodiment (Gibbs, 2005) is central to our understanding of the relationship between consciousness and the physical world. It is the idea that our experience and consciousness are not just the result of abstract processes in the brain, but are inherently linked to the physical body that contains and shapes these processes. The body provides a sensory interface through which we interact with the world and it acts as a filter and mediator of our experience. Our consciousness is not just an abstract representation of the world, but it is also a product of the way our body experiences the world. And even more so, consciousness could be no more than our embedded experience of the world.
On the contrary, the idea of a digital conscious AI, or the idea of transposing our consciousness into a digital universe, implies an absolutely radical dualism. It suggests that consciousness and the physical body can be completely separated, that our subjective experience can be reduced to algorithms and data structures. However, this view runs counter to the central idea of embodiment, which holds that our subjective experience is an embodied experience that is shaped and filtered by our physical body. In conclusion, the concept of embodiment suggests that our experience and consciousness are inherently linked to our body and that the idea of a digital conscious AI or transitioning consciousness into a digital universe, as it is presented in the short story sci-fi masterpiece “learning to be me” by Greg Egan, is incompatible with this idea. The subjective nature of qualia also makes it difficult to imagine how it could be modeled in-silico.
III. Language and knowledge
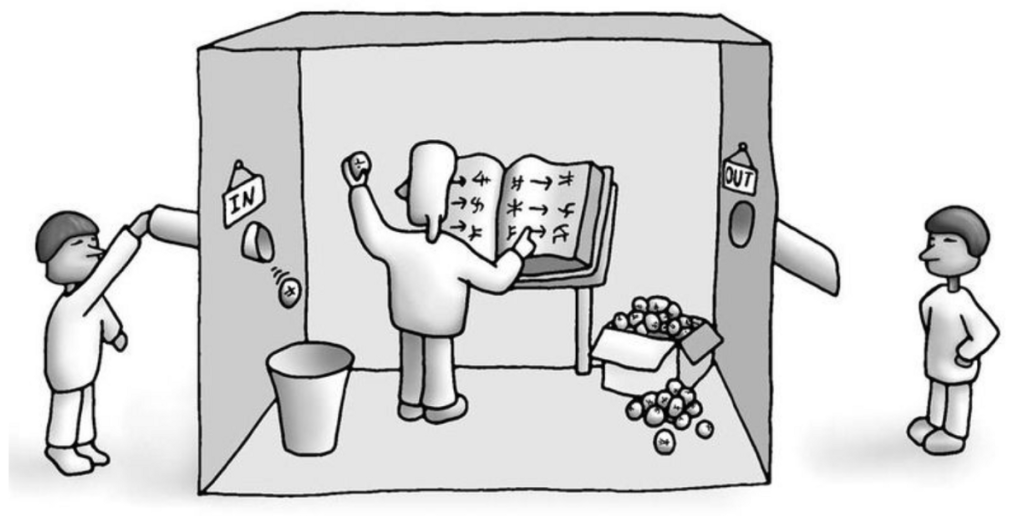
The Chinese Room argument, put forward by philosopher John Searle, is a thought experiment that challenges the idea of true artificial intelligence. It asserts that a machine, even one that can perform well on language tasks, does not truly understand language or have conscious thoughts and experiences. The experiment imagines a person in a room, who does not speak Chinese, receiving Chinese characters through a slot in the door and providing responses to questions based solely on rules provided by a book. The argument suggests that while the person in the room may be able to answer questions correctly, they do not understand the meaning of the language or the context in which it is used. It suggests that simply being able to process language does not equate to having a true understanding of language or the world. This challenges the view that AI can achieve true consciousness and understanding through purely computational processes and highlights the need for a deeper investigation into the nature of consciousness and intelligence.
The idea that knowledge can be reduced to language (Bakhtin, 1929; Barthes, 1953; Chomsky, 1965; Derrida, 1978; Eco, 1973; Wittgenstein, 1922) and that artificial intelligence (AI) can be created by manipulating symbols according to logical rules has been a dominant theme in philosophy and science. However, this view is limited by the fact that language is a low-bandwidth method of transmitting information and is often ambiguous, relying on non-linguistic understanding for clear communication. Meanwhile, recent advancements in language models, such as pre-trained language models (LLMs), have shown that language is inherently contextual and that the meaning of words and sentences is heavily influenced by the context in which they appear. However, given the limitations and difficulties of capturing the nuanced and context-sensitive nature of language and human understanding in models, some argue that the structural capture of language by vectorised models could, by itself, make an AI become self-aware as it is presented in the famous movie Her where the OS Samantha go towards the singularity.
The idea that knowledge is simply linguistic, and can be reduced to a collection of true propositions connected by logical rules, has a long history in philosophy and science. This view was influential in the development of Symbolic AI in the 20th century, where the focus was on manipulating symbols according to logical rules to produce intelligent behavior. In such a configuration it would be considered that knowing something simply means thinking the right sentence and grasping how it connects to other sentences in a big web of all the true claims we know. The ideal form of language, by this logic, would be a purely formal, logical-mathematical one composed of arbitrary symbols connected by strict rules of inference, but natural language could serve as well if you took the extra effort to clear up ambiguities and imprecisions. As Wittgenstein put it, “The totality of true propositions is the whole of natural science.” This position was so established in the 20th century that psychological findings of cognitive maps (Tolman, 1948) and mental images were controversial, with many arguing that, despite appearances, these must be linguistic at base.
In the latter half of the 20th century, this view began to be challenged as new findings in cognitive science and neuroscience highlighted that humans process information and make decisions through unconscious and embodied processes (Andler, 1994; Varela et al., 1992), such as perception, emotion, and motor control. It became clear that human intelligence is not reducible to a series of formal symbols or a set of rules, but rather is embodied in the physical and biological processes that make up the human organism (Varela et al., 1992). The idea that an AI’s knowledge consists solely of a database of true sentences connected by logical rules is now widely considered to be a narrow and limited view of intelligence. Research has shown that humans have a rich and complex internal representation of the world, which includes cognitive maps and mental images, and that this representation is not reducible to language alone. This has led to the development of alternative approaches to AI, such as connectionist and embodied AI, which seek to model not just the symbolic processing of language, but also the sensory and motor experiences that give rise to knowledge and intelligence in the first place.
The idea that an AI system can be considered intelligent if it manipulates symbols in the appropriate way, as proposed by the Turing test, is also coming under increasing scrutiny. While the ability to manipulate symbols is an important component of intelligence, it is not the only one, and does not capture the full richness and complexity of human experience. As Chomsky and his followers have argued for many years, language is not an effective and unambiguous means of communication. A significant portion of our knowledge is in fact nonlinguistic. This understanding is based on the observation that humans do not require a perfect means of communication to effectively understand each other (Christiansen, 2021). Our understanding of a sentence is often dependent on the context in which it is used, allowing us to make inferences about its meaning. This is evident in everyday conversations, where we often use contextual cues such as body language, tone of voice, and shared experiences to deepen our understanding of what is being communicated. The same holds true when reading a text, as comprehension depends on a deeper understanding of the topic being discussed, rather than solely relying on context-free reading strategies.
It’s interesting to note that humans acquire a lot of knowledge from nonlinguistic sources, such as direct exploration of the world, observation of the structures of artifacts, and participation in social customs and rituals. This type of knowledge often consists of nuanced patterns of information that are difficult to express in language, but are still accessible to us. For example, by exploring the world (Gopnik & Schulz, 2004), we learn about the behavior of objects and people, and the structures of artifacts and the environment convey information to us intuitively. Mental simulation (Constantinescu et al., 2016; O’Keefe & Dostrovsky, 1971) is another nonlinguistic way in which humans plan out scenarios and develop their skills, which can be used in crafting and reverse-engineering artifacts. Moreover, cultural knowledge is often passed on through precise movements and iconic representations (Descola, 2021), which are difficult to express in language but are still accessible to others.
“Large Language Models (LLMs) are a bit like mirrors : it gives the illusion of depth and can reflect almost anything, but it is only a centimeter thick. If we try to explore its depths, we bump our heads “
Yann Lecun
As pointed out by Yann Lecun and Jacob Browning in their article “AI and the limits of language”, all of this explains why a machine trained on language can know so much and yet so little. It is acquiring a small part of human knowledge through a tiny bottleneck. To capture language is only to capture the set of rules for manipulating the set of symbols that refer to the world in an incomplete way. It is thus a bit akin to a mirror: ”it gives the illusion of depth and can reflect almost anything, but it is only a centimeter thick. If we try to explore its depths, we bump our heads“. The analogy of a mirror is apt in this case, as it provides a superficial reflection of reality, but it does not capture the essence of what it reflects. Similarly, a machine trained on language can understand the words and phrases it has been exposed to, but it lacks the context and understanding that comes from a lifetime of experience and exposure to the world. This is why AI researchers are now focused on developing systems that can understand the world being discussed, rather than just the words themselves. The issue with LLMs is that they are limited to linguistic information, and there is no way to gain a deep understanding of the world solely through language. This highlights the limitations of language-based models and the need for AI systems to have a more comprehensive understanding of the world beyond just words. This, in turn, requires researchers to shift their focus away from language-based knowledge and towards embodied experience and world-knowledge.
IV. Intuition and knowledge
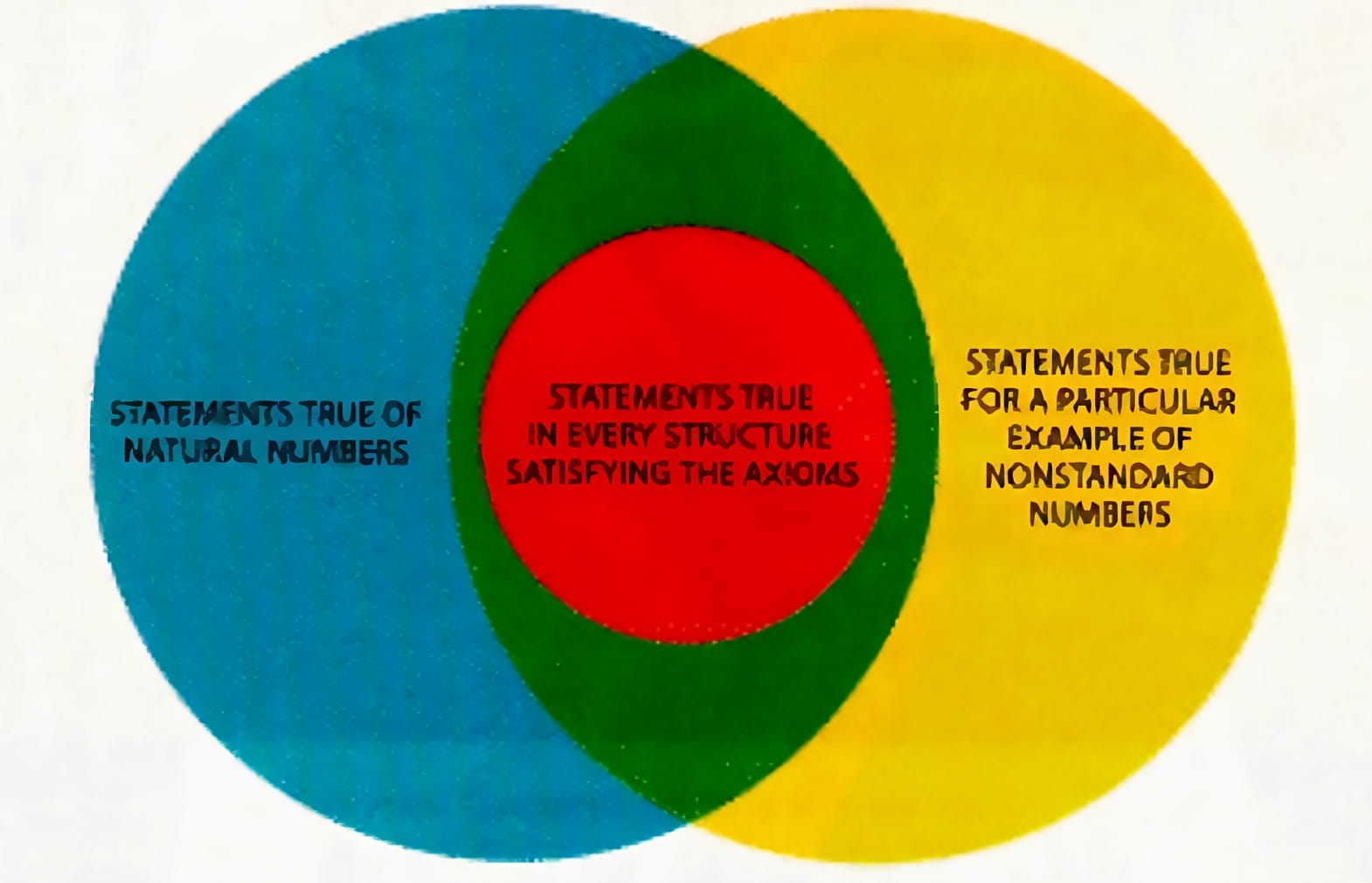
The Penrose-Lucas argument is a philosophical stance that suggests that human minds cannot be fully replicated in a machine. This argument is based on the combination of Kurt Gödel’s incompleteness theorems, Roger Penrose’s objections to computationalism, and John Lucas’s critique of artificial intelligence. Godel’s incompleteness theorem states that any system of axioms powerful enough to describe the arithmetic of whole numbers is inherently incomplete, meaning that there will always be true statements about the natural numbers that cannot be proven within the system. This theorem has important implications for our understanding of the limitations of formal systems, including computers and artificial intelligence.
In the context of AI, the incompleteness theorem highlights the difference between the kind of knowledge that can be captured by a formal system and the kind of knowledge that the human mind possesses. Penrose and Lucas highlight that the human mind is not a formal system, and our understanding of the world goes beyond what can be captured by any set of rules or algorithms. Our ability to think, reason, and make judgments is based on a rich tapestry of experiences, emotions, and intuition, which cannot be reduced to a set of formal rules. Therefore, it is not possible to create an AI system that is capable of fully replicating the human mind, since the human mind is not Turing-complete. Godel’s incompleteness theorem shows us that there will always be aspects of human understanding that cannot be captured by a formal system, and that there will always be limits to what AI can achieve.
To truly create AI systems that can match or surpass human intelligence and look for AGI, researchers insist on the importance of incorporating human values, ethics, and intuition into the development of AI systems, to ensure that they are aligned with human interests and serve our well-being. Researchers must find ways to embody AI with sensory and motor experiences, and to develop models that can capture the full range of human cognition, from perception and action to reason and introspection as we assist to a paradigm shift in Cognitive AI with the development of human like common sense(Zhu et al., 2020).
Consider the phrase ‘The falling rock smashed the bottle’. To understand this sentence, you have to know what a rock is and what it means for something to fall. But to understand what a rock is, you have to know what an object is. To grasp what falling is, you have to understand motion and space. And to understand the relationship between falling and smashing, you have to understand causality. As it was demonstrated by Kan(Kant, 1781; Strawson & Allais, 2018), indeed, an understanding of objects, motion, space, and causality is a prerequisite for understanding any aspect of the everyday world, not just falling and rocks. Unfortunately, this foundational layer of common sense, which is a prerequisite for human-level intelligence, is lacking in today’s AI systems.
One way to approach embodied Artificial Intelligence is to explore the possibility of developing unsupervised action-based world models (Zhu et al., 2020). The idea is to replicate the way humans develop a model of the world through experiencing it. The way humans experience the world is rooted in their bodies, and the movements, actions and sensations they encounter. One approach to embodied AI is to build robots or other physical systems that can interact with the world in a similar way to humans, or animals in general (Shanahan et al., 2020), by having sensors and actuators to perceive and act in the environment. These systems can then be trained using unsupervised learning methods, where they learn from the raw sensory data they receive and the actions they take in response, without being explicitly programmed with any particular task or goal. In this way, embodied AI systems can develop their own understanding of the world, and develop their own way of performing tasks that are relevant to the environment they are in. This is in contrast to traditional AI systems that are trained on pre-existing data and are not able to interact with the world in a dynamic way.
The idea of in silico consciousness emerging from embodied AI is based on the hypothesis of emergence (Edelman & Tononi, 2008; Watanabe et al., 2011), which suggests that complex systems can exhibit novel properties that cannot be predicted from the properties of their individual components. According to this view, consciousness could emerge as a result of the interactions between various components in an embodied AI system, such as sensors, actuators, and algorithms. The lack of intuitive understanding of the physical world, efficient learning, transfer learning, imagination and planning, among other things, all contribute to the limitations of an unsupervised world action-based model from which could emerge a general intelligence. But ultimately, the pursuit of creating consciousness in AI may serve less as a goal in itself than as a tool for better understanding the human brain and its workings (Hassabis et al., 2017; Khaligh-Razavi & Kriegeskorte, 2014). Through the process of distilling intelligence into an algorithmic construct and comparing it to the human brain, we may gain insights into some of the most profound mysteries of the mind such as creativity, dreams, and perhaps one day, even consciousness.
Conclusion
In conclusion, the journey to understand the emergence of sentience in machines is an ongoing and complex task. It is evident that language-based models such as LLMs have limitations in grasping the nuances of human knowledge and understanding of the world. The Godel’s incompleteness theorem highlights the limitations of formal systems, including machines, in capturing the entirety of mathematical truth or knowledge in general. Similarly, the inability of LLMs to simulate non-linguistic mental simulation, social customs, and physical world knowledge presents a major gap in their capacity to truly understand the world. These limitations make it apparent that AI systems that strive to achieve true sentience must go beyond the acquisition of language and knowledge through a narrow bottleneck. Instead, they must focus on developing a deep understanding of the world through physical and social experiences, just as humans do. The task at hand for AI, neuroscientists, psychologues and cognitive science researchers is to find ways to bridge these gaps in understanding and pave the way for machines to truly comprehend the world through understanding better and modeling the sense of self, introspection, meta cognition, intuition or imagination.
References
Anderson, J. R., Bothell, D., Byrne, M. D., Douglass, S., Lebiere, C., & Qin, Y. (2004). An Integrated Theory of the Mind. Psychological Review, 111, 1036‑1060. https://doi.org/10.1037/0033-295X.111.4.1036
Andler, D. (1994). Introduction aux Sciences Cognitives. Folio.
Arcas, B. A. y. (2022, février 16). Do large language models understand us? Medium. https://medium.com/@blaisea/do-large-language-models-understand-us-6f881d6d8e75
Bakhtin. (1929). Problems of Dostoevsky’s Art.
Bar-Hillel, Y. (s. d.). A demonstration of the non-feasibility of fully-automatic high-quality machine translation.
Barthes, R. (1953). Le Degré zéro de l’écriture.
Bender, E. M., & Koller, A. (2020). Climbing towards NLU : On Meaning, Form, and Understanding in the Age of Data. Proceedings of the 58th Annual Meeting of the Association for Computational Linguistics, 5185‑5198. https://doi.org/10.18653/v1/2020.acl-main.463
Bisk, Y., Holtzman, A., Thomason, J., Andreas, J., Bengio, Y., Chai, J., Lapata, M., Lazaridou, A., May, J., Nisnevich, A., Pinto, N., & Turian, J. (2020). Experience Grounds Language. Proceedings of the 2020 Conference on Empirical Methods in Natural Language Processing (EMNLP), 8718‑8735. https://doi.org/10.18653/v1/2020.emnlp-main.703
Bransford, J. D., & Johnson, M. K. (1972). Contextual prerequisites for understanding : Some investigations of comprehension and recall. Journal of Verbal Learning and Verbal Behavior, 11(6), 717‑726. https://doi.org/10.1016/S0022-5371(72)80006-9
Bratton, B. (2022). The Model Is The Message. https://www.noemamag.com/the-model-is-the-message
Browning, J. (2021). Making Common Sense. https://www.noemamag.com/making-common-sense
Browning, J. (2022). AI And The Limits Of Language. https://www.noemamag.com/ai-and-the-limits-of-language
Chomsky, N. (1965). Aspects of the Theory of Syntax (50e éd.). The MIT Press. https://www.jstor.org/stable/j.ctt17kk81z
Christiansen, morten. (2021). The Language Game. https://www.basicbooks.com/titles/morten-h-christiansen/the-language-game/9781541674981/
Claverie, B. (2019). Dynamique exponentielle et naturalité de l’intelligence artificielle. Hermès, La Revue, 85(3), 187‑200. https://doi.org/10.3917/herm.085.0187
Cole, D. (2020). The Chinese Room Argument. In E. N. Zalta (Éd.), The Stanford Encyclopedia of Philosophy (Winter 2020). Metaphysics Research Lab, Stanford University. https://plato.stanford.edu/archives/win2020/entries/chinese-room/
Daston, & Galison. (2010). Objectivity. https://press.princeton.edu/books/paperback/9781890951795/objectivity
Daws, R. (2020, octobre 28). Medical chatbot using OpenAI’s GPT-3 told a fake patient to kill themselves. AI News. https://www.artificialintelligence-news.com/2020/10/28/medical-chatbot-openai-gpt3-patient-kill-themselves/
Delacour, J. (1995). An introduction to the biology of consciousness. Neuropsychologia, 33(9), 1061‑1074. https://doi.org/10.1016/0028-3932(95)00048-8
Derrida, J. (1978). Cogito and the History of Madness.
Descola, P. (2021). Les formes du visible. In Http://journals.openedition.org/lectures. Seuil. https://journals.openedition.org/lectures/54893
Eco, U. (1973). Signo.
Edelman, G. M., & Tononi, G. (2008). A Universe Of Consciousness : How Matter Becomes Imagination. Hachette UK.
Egan, G. (s. d.). Learning To Be Me. Consulté 4 février 2023, à l’adresse https://www.goodreads.com/book/show/53144942-learning-to-be-me
Frege, G. (1956). The Thought : A Logical Inquiry. Mind, New Series, 65(259), 289‑311.
Gamez, D. (2018). Human and Machine Consciousness (1re éd.). Open Book Publishers. https://www.jstor.org/stable/j.ctv8j3zv
GANASCIA, J.-G. (2017). Le mythe de la Singularité, Faut-il craindre l’intelligence artificielle ? ÉDITIONS DU SEUIL.
Gibbs, R. W. (2005). Embodiment and Cognitive Science. Cambridge University Press.
Gopnik, A., & Schulz, L. (2004). Mechanisms of theory formation in young children. Trends in Cognitive Sciences, 8(8), 371‑377. https://doi.org/10.1016/j.tics.2004.06.005
Hassabis, D., Kumaran, D., Summerfield, C., & Botvinick, M. (2017). Neuroscience-Inspired Artificial Intelligence. Neuron, 95(2), 245‑258. https://doi.org/10.1016/j.neuron.2017.06.011
Ivanova, A. A., & Mahowald, K. (2022, juin 24). Google’s powerful AI spotlights a human cognitive glitch : Mistaking fluent speech for fluent thought. The Conversation. http://theconversation.com/googles-powerful-ai-spotlights-a-human-cognitive-glitch-mistaking-fluent-speech-for-fluent-thought-185099
Jonas, E., & Kording, K. P. (2017). Could a Neuroscientist Understand a Microprocessor? PLOS Computational Biology, 13(1), e1005268. https://doi.org/10.1371/journal.pcbi.1005268
Kant, E. (1781). Critics of Pure Reason.
Khaligh-Razavi, S.-M., & Kriegeskorte, N. (2014). Deep Supervised, but Not Unsupervised, Models May Explain IT Cortical Representation. PLoS Computational Biology, 10(11), e1003915. https://doi.org/10.1371/journal.pcbi.1003915
Le Blanc, B., & Claverie, B. (2016a). Entre sensation et cognition : L’illusion explicative. Hermès, La Revue, 74(1), 66‑70. https://doi.org/10.3917/herm.074.0066
Le Blanc, B., & Claverie, B. (2016b). La perspective artificielle des sens. Hermès, La Revue, 74(1), 183‑187. https://doi.org/10.3917/herm.074.0183
Levesque, H. J. (s. d.). The Winograd Schema Challenge.
Marcus, G. (2022, mars 10). Deep Learning Is Hitting a Wall. Nautilus. https://nautil.us/deep-learning-is-hitting-a-wall-238440/
Millière, R. (2022, juillet 2). Moving Beyond Mimicry in Artificial Intelligence. Nautilus. https://nautil.us/moving-beyond-mimicry-in-artificial-intelligence-238504/
Moyer, E. (2018, mars 7). Yes, Alexa is suddenly letting out evil cackles for no reason. CNET. https://www.cnet.com/home/smart-home/yes-amazon-alexa-is-randomly-laughing-for-no-reason/
Neo, L. B.-L., CEDS, Casper. (2021, décembre 15). Anthropomorphizing the Algorithm : Animism in the Age of AI. ACEDS. https://aceds.org/anthropomorphizing-the-algorithm-animism-in-the-age-of-ai/
Nguyen, B. (s. d.). Suspended Google engineer says the AI he believes to be sentient hired a lawyer. Business Insider. Consulté 4 février 2023, à l’adresse https://www.businessinsider.com/suspended-google-engineer-says-sentient-ai-hired-lawyer-2022-6
Pathways Language Model (PaLM) : Scaling to 540 Billion Parameters for Breakthrough Performance. (2022, avril 4). https://ai.googleblog.com/2022/04/pathways-language-model-palm-scaling-to.html
Reboul, A. (2015). Why language really is not a communication system : A cognitive view of language evolution. Frontiers in Psychology, 6. https://www.frontiersin.org/articles/10.3389/fpsyg.2015.01434
Roberts, W. A. (s. d.). Mental Time Travel : Animals Anticipate the Future. https://doi.org/DOI: 10.1016/j.cub.2007.04.010
Shallice, T. (1988). From Neuropsychology to Mental Structure. Cambridge University Press.
Shanahan, M., Crosby, M., Beyret, B., & Cheke, L. (2020). Artificial Intelligence and the Common Sense of Animals. Trends in Cognitive Sciences, 24(11), 862‑872. https://doi.org/10.1016/j.tics.2020.09.002
Smith, B. C. (s. d.). What’s Still Lacking in Artificial Intelligence. Scientific American Blog Network. Consulté 4 février 2023, à l’adresse https://blogs.scientificamerican.com/observations/whats-still-lacking-in-artificial-intelligence/
Stephen T Asma, A. (2020, mars 8). Our growing intimacy with machines is rooted in the ancient practice of animism [Text]. Scroll.In; https://scroll.in. https://scroll.in/article/954824/our-growing-intimacy-with-machines-is-rooted-in-the-ancient-practice-of-animism
Strawson, P., & Allais, L. (2018). The Bounds of Sense : An Essay on Kant’s Critique of Pure Reason. Routledge. https://doi.org/10.4324/9780429447075
The Evolved Apprentice « On the Human. (s. d.). Consulté 4 février 2023, à l’adresse https://nationalhumanitiescenter.org/on-the-human/2010/08/the-evolved-apprentice/
Turing, A. M. (2009). Computing Machinery and Intelligence. In R. Epstein, G. Roberts, & G. Beber (Éds.), Parsing the Turing Test (p. 23‑65). Springer Netherlands. https://doi.org/10.1007/978-1-4020-6710-5_3
Tye, M. (2000). The Imagery Debate. https://mitpress.mit.edu/9780262700733/the-imagery-debate/
Varela, F. J., Thompson, E. T., & Rosch, E. (1992). The Embodied Mind : Cognitive Science and Human Experience (Revised ed. edition). The MIT Press.
Waal, F. de. (2016, avril 8). Opinion | What I Learned From Tickling Apes. The New York Times. https://www.nytimes.com/2016/04/10/opinion/sunday/what-i-learned-from-tickling-apes.html
Wexler, N. (s. d.). Why We’re Teaching Reading Comprehension In A Way That Doesn’t Work. Forbes. Consulté 4 février 2023, à l’adresse https://www.forbes.com/sites/nataliewexler/2019/01/23/why-were-teaching-reading-comprehension-in-a-way-that-doesnt-work/
Wittgenstein, L. (1922). Tractatus Logico-Philosophicus.
Zhu, Y., Gao, T., Fan, L., Huang, S., Edmonds, M., Liu, H., Gao, F., Zhang, C., Qi, S., Wu, Y. N., Tenenbaum, J. B., & Zhu, S.-C. (2020). Dark, Beyond Deep : A Paradigm Shift to Cognitive AI with Humanlike Common Sense. Engineering, 6(3), 310‑345. https://doi.org/10.1016/j.eng.2020.01.011