In 2019, the National Health Service (NHS) teamed up with Amazon to provide elderly people, blind people and other patients with Alexa, an AI-powered voice assistant that aids these patients in retrieving NHS verified health advice [1]. With this approach, the NHS aims to relieve the workload of healthcare employees. While AI seems to take on the role of an assistant in this case, over the years attempts have been made to make AI systems fully autonomous. An example of this is the Full Self-Driving (FDS) feature that Tesla is currently developing [2]. Imagine you are sitting on the driver’s seat with your hands off the steering wheel. Your tesla is driving on a busy urban road. What could possibly go wrong? On the 24th of November 2022, an eight-vehicle crash happened [3]. The cause of this crash was a tesla abruptly braking whilst changing lanes. This accident left nine people injured, including a two-year-old child and blocked traffic for more than an hour. While AI decision making can certainly be beneficial in certain cases, it may lead to disastrous consequences in others. In a world where humans become increasingly dependent on AI, how far should we go in delegating human decision making to AI?
In this essay, we argue that human supervision of AI decision-making is crucial to limit possible disastrous outcomes of choices made by an AI system.
Questioning the reliability of AI systems
AI systems like the self-driving cars from tesla will become an essential part of our lives. However, these seemingly “perfect” AI systems contain controversial biases which can result in a lack of fairness, questioning the reliability of the systems. AI bias takes various forms such as gender bias, racial prejudice or age discrimination etc. For example, an AI system called COMPAS was used throughout the US judicial court system for predicting the likelihood of criminals re-offending. The COMPAS tool assigned defendants a score from 1 to 10. These scores profoundly affected the lives of the defendants as they determined their detainment. However, as ProPublica and The Washington Post pointed out that an implemented AI system contained major biases towards black people [4]. Even though the COMPAS tool excludes race attributes in their decision making, it still predicted a two times higher likelihood for black defendants to re-offend, while these defendants did not go out and committed another crime. These examples illustrated the possibly dangerous and discriminatory consequences which undermine equality and oppress minorities if we let AI dictate essential decision-making.
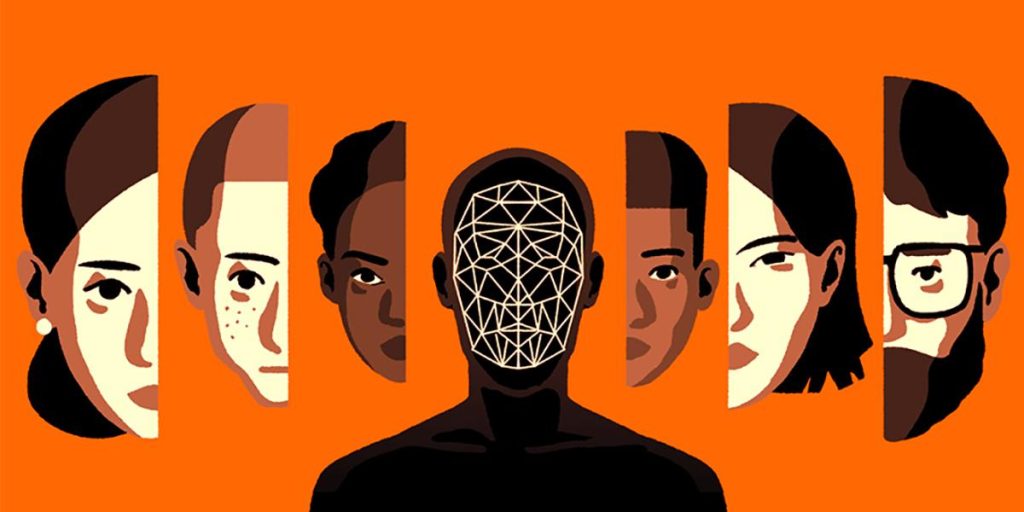
Over the years we have become aware of biases in AI systems. Since then, researchers have increased efforts to crack the AI system and offer explainability. Still, current research has reached limited success of opening the “black box”. The issue of explainability becomes even more apparent with a recently published paper showing that AI researchers and developers increasingly struggle to explain how complex AI systems work [5]. This lack of explainability and transparency of “black box” AI systems makes it impossible to understand the causes of failure and improve system safety. It is imperative to acknowledge that relying on AI systems for decision making is not prudent, as the inability to assess their reliability and evaluate the potential harm undermines their trustworthiness.
AI and accountability: an even more complicated matter
AI accountability is a crucial issue in the development and deployment of artificial intelligence. With AI systems becoming more prevalent and having greater impact on society, it’s imperative that they be used responsibly and ethically. This section focuses on the accountability gap in AI decision-making and draws insights from two articles: “The accountability gap in AI decision-making” and “AI’s accountability problem” [6][7].
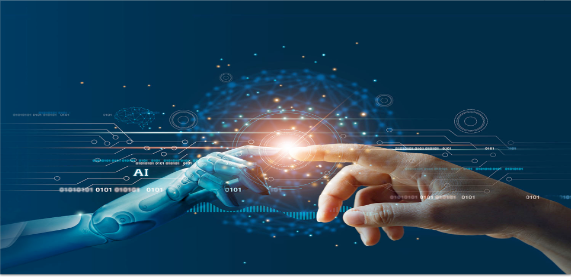
In “The accountability gap in AI decision-making,” Crootof highlights the limitations of current legal and regulatory frameworks in ensuring accountability for AI systems. She notes that AI decisions can have far-reaching consequences, and the lack of transparency in these systems makes it difficult to attribute cause and effect. Current frameworks are often not equipped to handle the unique challenges posed by AI, leading to a significant accountability gap.
Hao’s “AI’s accountability problem” highlights the challenges of ensuring accountability in AI systems. The lack of transparency and difficulty in attributing cause and effect make it difficult to hold AI systems accountable. Hao notes that these challenges are compounded by the fact that AI systems can make decisions with wide-ranging impacts and there is often no clear way to hold them accountable.
To close the accountability gap in AI, new legal and regulatory frameworks must be developed specifically to address the challenges posed by AI decision-making. This could include the creation of accountability mechanisms for AI systems, such as an AI ombudsman or a dedicated AI regulatory body. Additionally, ethical considerations must be incorporated into AI development and decision-making processes of organizations and governments to ensure responsible and ethical use of AI.
Finally, ensuring accountability in AI decision-making is crucial for maximizing its benefits and minimizing negative impacts. By focusing on the accountability gap in AI decision-making, this section highlights the limitations of current frameworks and possible solutions to ensure responsible and ethical use of AI. It is up to organizations and governments to take action and close the accountability gap in AI decision-making.
Morality and the lack thereof in AI systems
Many decisions made by humans are rooted in human values. Consider the trolley problem in the figure below:
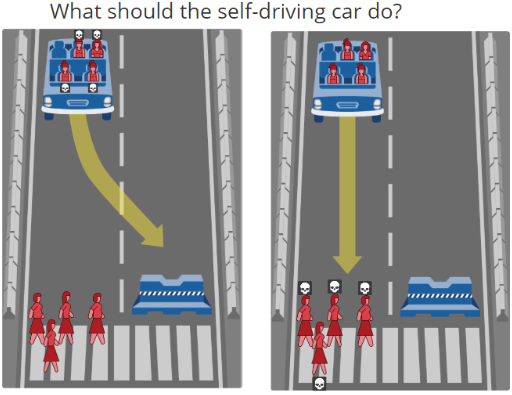
A self-driving car is about to crash into four people crossing over the road. The car has the opportunity to avoid these four people by switching to the left lane. However, in doing so, the passengers will be sacrificed, as the car will crash into a roadblock, killing the passengers. Should the self-driving car switch to the left lane? In this scenario, there is no right or wrong answer. The answer you choose is based on your upbringing, your experiences and most importantly your values. Another example in which human values are crucial is the implementation of positive discrimination. In a corporate job that is dominated by men, you seek to employ more women to balance out the gender ratio. In this case, one needs to be aware that women are underrepresented in that particular occupation, and that this is a bad thing. The current AI systems struggle to make decisions that are not black and white.
To start with, these decisions require a certain level of subjectivity, while AI relies on data that it is fed, which means that in a way, if bias is not considered, AI is purely objective. Subjectivity means that multiple decisions are possible, so it depends on the human to decide what decision is appropriate according to them. Therefore, human supervision of AI decision-making, in this context, is inevitable.
As AI relies on data that it is fed, another concern that needs to be raised again is bias. If bias is considered and it does exist in the data that is being fed to the AI, there is a possibility that the machine could make an unethical decision. One example of this can be reviewed in the section in which the reliability of the AI systems is questioned.
Finally, humans have attributes that are crucial for making decisions that are morally grey, and these attributes are hard to teach AI systems. One example of such an attribute is empathy, which is the ability to understand emotions. In healthcare, empathy is essential in clinical scenarios. Montemayor, Halpern, and Fairweather conclude in their paper about replacing human empathy by AI systems in healthcare that this replacement is impossible because an AI cannot feel genuine empathy, and this replacement is unethical because using AI in these situations can erode the expectations of real human empathy, which human beings in distress do not deserve [8].
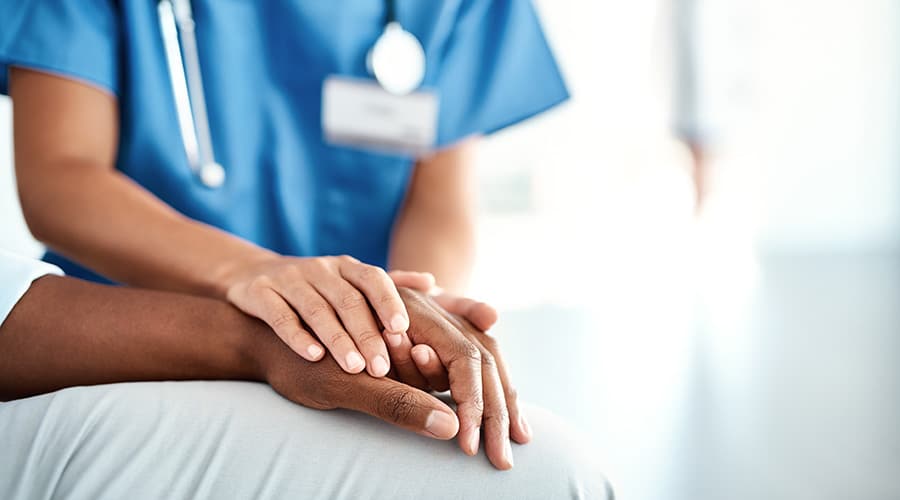
Conclusion
To summarize, delegating the decision making by humans to machines, unsupervised, raises important concerns about the reliability behind AI systems, as they still seem to suffer from bias and lack of explainability, undermining the trustworthiness of AI. Another concern that deserves attention is the accountability gap in AI decision-making, which is demonstrated by the limitations of current frameworks and possible solutions to ensure responsible and ethical use of AI. Finally, the difficulty in AI taking decisions about matters that can be seen as morally grey is addressed, as such decisions tend to be subjective, making human supervision inevitable, and the decisions require certain human qualities that are hard to reconstruct artificially, such as empathy. To conclude, given the concerns that AI systems still suffer from, it is important that humans supervise machines to limit possible disastrous outcomes of choices. In other words: AI, big brother is watching you.
References
- Siddique, H.: Nhs teams up with amazon to bring alexa to patients (Jul 2019),
https://www.theguardian.com/society/2019/jul/10/nhs-teams-up-with-amazon-to-
bring-alexa-to-patients - Pisharody, G., Ponnezhath, M.: Tesla’s full self-driving beta now available to all
in n. america, musk says (Nov 2022), https://www.reuters.com/business/autos-
transportation/teslas-full-self-driving-beta-now-available-all-n-america-musk-says-
2022-11-24 - Klippenstein, K.: Exclusive: Surveillance footage of tesla crash on sf’s bay
bridge hours after elon musk announces ”self-driving” feature (Jan 2023),
https://theintercept.com/2023/01/10/tesla-crash-footage-autopilot/ - Sam Corbett-Davies, Emma Pierson, A.F., Goel, S.: A computer program used for
bail and sentencing decisions was labeled biased against blacks. it’s actually not that
clear., https://www.washingtonpost.com/news/monkey-cage/wp/2016/10/17/can-an-
algorithm-be-racist-our-analysis-is-more-cautious-than-propublicas/ - Samados, A., Aggarwal, N., Cowls, J., Morley, J., Roberts, H., Taddeo, M., Floridi, L.: The ethics of algorithms: key problems and solutions. Ethics, Governance, and Policies in Artificial Intelligence pp. 97–123 (2021)
- Hao, K.: Congress wants to protect you from biased algorithms, deepfakes, and other
bad ai (Apr 2020), https://www.technologyreview.com/2019/04/15/1136/congress-
wants-to-protect-you-from-biased-algorithms-deepfakes-and-other-bad-ai/ - Crootof, R.: Ai and the actual ihl accountability gap (Dec 2022), https://papers.ssrn.com/sol3/papers.cfm?abstractid = 4289005
- Montemayor, C., Halpern, J., Fairweather, A.: In principle obstacles for empathic ai:
Why we can’t replace human empathy in healthcare. AI & Society 37(4), 1353–1359
(2021). https://doi.org/10.1007/s00146-021-01230-z