
On January 9, 2020, Robert Julian-Borchak Williams was called by the Detroit Police Department with the request to turn himself in. Williams assumed this was a silly joke, but an hour later the police showed up at his house to arrest him in front of his wife and children. After spending a night in jail, he was told he was suspected of stealing $3,800 worth of watches. One of the detectives slid a photo from the jeweler’s security camera under Williams’ nose, showing a black man with a cap. The detective asked him if he was the man in the photo, but it was clear he wasn’t. Williams’ arrest turned out to be the consequence of a mismatch in an AI facial recognition algorithm due to a racial bias. Unfortunately, this was not a one-off incident as it appears that AI models are vulnerable to develop biases (e.g. Amazon’s AI recruiting tool, Google’s photo app, Microsoft’s AI chatbot, and AI platform COMPAS). This is worrying, as the widespread use of these biased models can cause inequality to rise. An additional problem is that these models are not transparent due to their complexity and that the legal tools are lacking to hold companies responsible for the functioning of the models. Nonetheless, AI models are used increasingly more in corporate and government decision-making. This makes it all the more complicated to filter out unethical AI models to ensure people like Robert Williams are never wrongfully arrested again. To prevent such cases in the future, companies started drawing up ethical frameworks and AI ethics boards en masse. They seemed to see the seriousness of the problem and this positive development was welcomed with applause. However, a closer look at these frameworks shows that they do not function well because the guidelines are imprecise and biases persist. It seems that the companies cannot take responsibility for creating ethical AI. It is time for appropriate regulation of AI that entails a change in culture.
The Problems with Ethical Frameworks
In practice, we see that the ethical frameworks and the AI ethics boards do not deliver the desired results and that there are quite a few shortcomings. A common criticism is that the frameworks mainly consist of vague guidelines that are not quantified. Bombastic words are often used in these guidelines, such as fairness and transparency, which mainly appeal to the outside world. However, due to the lack of concrete requirements, the guidelines are difficult to implement.
A second criticism is that the AI ethics programs of Big Tech companies are not transparent, according to Rumman Chowdhury. Chowdhury, lead for responsible AI at Accenture, points out that it is unclear whose interests are represented by these companies’ AI ethics boards. This is in contrast to the ethics councils of other socially impactful institutions, such as universities and hospitals, where it is clear that they represent the interest of the general public. In addition, Chowdhury indicates that it is often unclear when ethics boards intervene and what is done about the problem. In an interview with The Verge she uses the AI ethics board of Google as an example: “This board cannot make changes, it can just make suggestions. They can’t talk about it with the public. So what oversight capabilities do they have?” An example illustrating the ambiguity about the influence of these AI ethics councils comes from Microsoft. Eric Horvitz, Microsoft’s top researcher, said the company has lost significant revenue because they followed the advice of their ethics committee, Aether. What this advice actually was about, however, is not made public. Due to the lack of transparency, it is therefore not possible to understand where Microsoft draws the line for unethical use of AI.
“This board cannot make changes, it can just make suggestions. They can’t talk about it with the public. So what oversight capabilities do they have?”
Rumman Chowdhury
Then we arrive at the last point of criticism, which is a continuation of the previous two points, namely accountability. It raises the question whether we can actually trust companies to self-regulate. Will they hold themselves accountable and make meaningful changes when flaws are discovered? This is a question of conscience. However, there are examples from the past that tend to answer this question negatively. In 2015, software engineer Jacky Alciné disclosed that his black friends were classified as “gorillas” by Google’s photo app that uses AI facial recognition technology. Three years later, in 2018, Google appeared to have ‘fixed’ this problem by simply removing the “gorilla” label. This does not indicate that the original problem has been solved, but rather that a quick-fix has been chosen. Can this be considered a successful example of self-regulation? Another example is that of researcher Timnit Gebru, who was fired from Google without explanation. In her past, Gebru has been critical of the lack of minorities at Google and she exposed the biases of Google’s search engine. Gebru was never given an explanation for her release but she is convinced it had to do with her criticism. In this criticism, Gebru was supported by researcher Margaret Mitchell, who also had to leave Google. How can we trust a tech giant like Google if they fire their own employees for being critical and pointing out proven ethical flaws in their system?
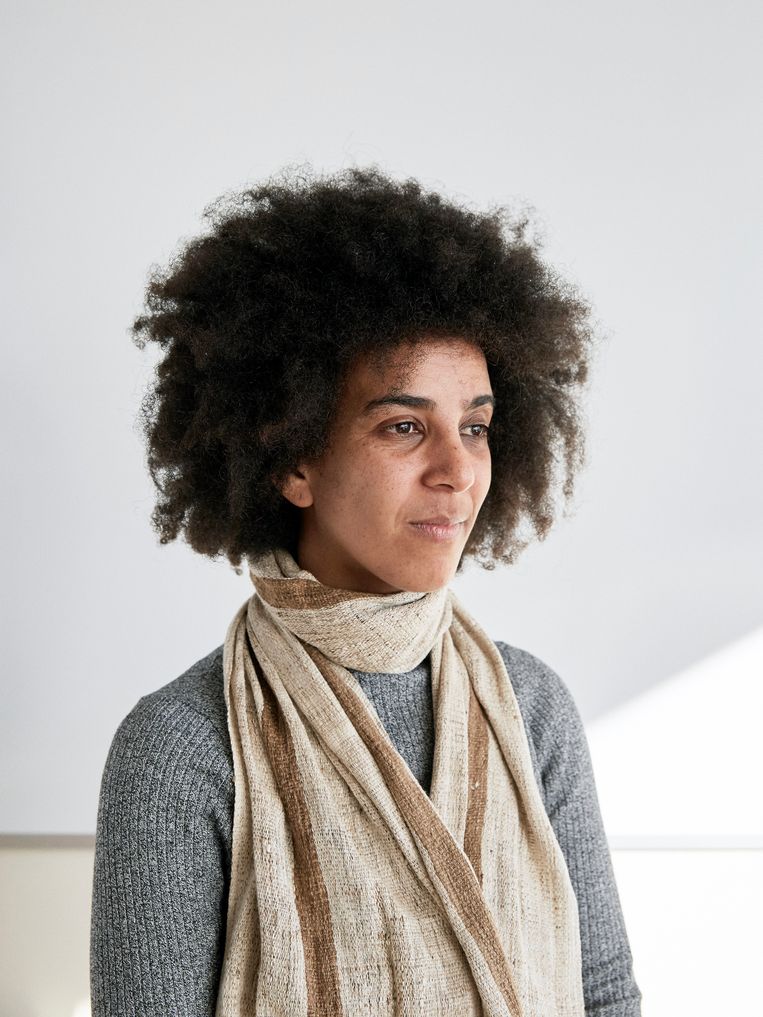
Ethics Washing: Exposing a Problematic Culture
The critical points discussed above are problematic for the development of ethical AI. To the outside world, the companies seem to be very committed to the development of ethical AI. However, in practice, there are many snags. This creates the illusion that the weaknesses of AI models are getting resolved. But why would companies spend all that time, money, and effort developing these ethical frameworks if the implementation doesn’t turn out to be a high priority? What’s in there for them? To begin with, these ethical frameworks are excellent for a company’s reputation and they can be used positively for marketing campaigns. But according to researcher Ben Wagner, there is more behind these ethical frameworks than just a nice marketing tool. Wagner calls tech companies’ enthusiasm for AI ethics nothing more than “ethics washing”, a method of evading government regulation.

Ethics washing, also called ethics theater, is “the practice of fabricating or exaggerating a company’s interest in equitable AI systems that work for everyone”. According to Wagner, setting up an ethical framework is the perfect deflection mechanism for companies when confronted with biases in their technologies, because they can point to their framework and say they are doing something about the problem. In addition, Wagner says that this deflection mechanism, together with the fact that the AI ethics committees have no power to enforce their advice, ensures that nothing is done about the issues. These ethical frameworks also prevent governments from regulating AI, which is in the interest of the companies. For example, if all AI models developed by companies were subject to strict government regulations, then there would be a higher probability that flaws will be found in the model. This could prevent some of these models from going into production, leading to lost profits. Besides, when a company makes its own decisions about the ethics of its AI models, it can accelerate the model development and make its own decisions about ethical validity, without anyone else looking over its shoulder.
Call to Action
The likes of Chowdhury, Gebru, and Wagner expose a deep-seated cultural problem in which a combination of questionable ethical awareness and lack of government oversight leads to a system in which companies fail to self-regulate. So it is clear that something needs to change in order to keep AI safe for everyone. But how are we going to realize this change? We discuss three points to get rid of ethics washing and create an environment that prioritizes AI ethics.
First of all, the gap between AI and lawmakers is too big. This is an often mentioned point of criticism from opponents of government oversight, as lawmakers would lack the knowledge to establish regulations for AI. A painful example of this was Mark Zuckerberg’s interrogation in front of Congress regarding the oversight of Facebook. During the hearing, it became clear that lawmakers often had no idea what they were talking about when it comes to technology. To fill this gap in the short term, it is important that lawmakers are familiarized by AI experts when drafting legislation. However, in the long run, it is crucial that lawmakers become less reliant on AI experts’ knowledge so that they can legislate critically and avoid regulation that only promotes AI experts’ objectives.

As a result, future lawmakers, specializing in technology law, should have a basic understanding of how AI models work, how these models are technically developed, and which implementations are viable. This could be achieved, for example, by making it compulsory for law students to take a few AI courses or a minor in AI – with an emphasis on the technical side of AI – before they are eligible for a specialization in technology law. In this way, their understanding of the workings of AI models will increase, which allows them to create legal tools to regulate AI effectively and independently. Next to that, this would also make lawmakers act faster and prevent them from lagging behind on the rapid development of AI, which is an often-used argument against regulation proposed by opponents.
[…] it is crucial that lawmakers become less reliant on AI experts’ knowledge so that they can legislate critically and avoid regulation that only promotes AI experts’ objectives.
Secondly, the ethical frameworks must consist of measurable principles, because at the moment there are no tools for developers to ensure that the models are ethical. Critics have argued that ethical principles, such as fairness and privacy, cannot be quantified because the meaning is different for everyone. However, many of these abstract principles have already been quantified using a combination of research, legal precedents, and technical best practices. Privacy, for example, is an important topic in AI ethics and there are multiple ways to actually quantify possible privacy violations such as the use of k-anonymity. Here a dataset is called k-anonymous (where k is a predefined number) if, for any record of a person in the dataset, there are at least k-1 other records that are indistinguishable. These kinds of tacit requirements allow us to concretely examine models on ethicality.
Finally, and perhaps most crucially, AI students and students in allied fields must be properly educated on ethics. A government can regulate whatever it wants, but if AI experts do not believe in the idea of ethical AI then it will, in any case, stay difficult to have healthy AI development. Therefore, a change in mind and culture is needed. While these issues arise in all kinds of companies and even governments, this cultural change must be initiated at the root, namely in the university programs that teach AI. At the moment, only 18% of the data science students learn about the ethics of AI, which is alarming, to say the least. Next to that, in most of the cases where ethics do play a role in the curriculum, these are often only summarized in one course. However, as most AI technologies that are taught include ethical considerations, we argue that instead of just one course, ethics should be an integral part of every stage of learning AI.
All in All…

The role of AI in our daily lives is growing rapidly and with the current ethics programs in use, those whose lives it was supposed to improve are being disadvantaged. The fact that Big Tech companies are not transparent about their ethics programs and the way these are implemented makes it only more difficult. And most of all, while it is the AI companies that are required to act ethically, it’s strange that they are also the ones that are regulating the AI frameworks in the first place. It’s as if someone is making the rules of a game while playing it at the same time. Therefore, something has to radically change. The gap between lawmakers and AI needs to be filled by education and vague ethical guidelines need to be transformed towards tangible, measurable guidelines. The most essential change, though, is a cultural shift. AI experts need to understand the importance of ethics in every field of AI and ethics needs to play an integral part in AI education for that. Because without the right mindset no ethical framework will make the difference.