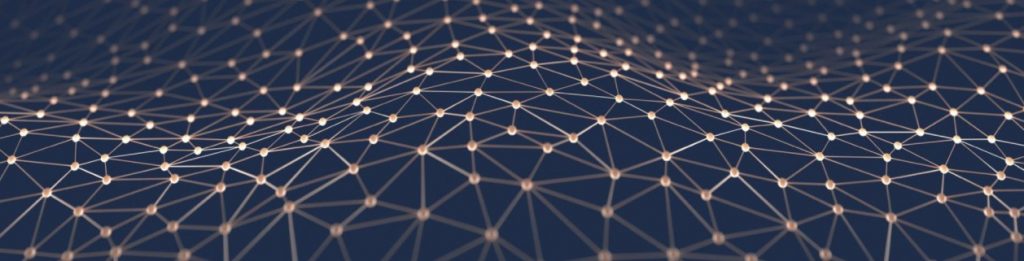
Artificial Intelligence (AI) is more present than ever in our daily lives. Whether you open your phone with a face id, watch your favorite series on Netflix or check your social media, AI is involved. Besides the involvement of AI in our personal lives, AI is taking over the bigger industries, such as transportation, retail, financial services and even healthcare. AI has made its appearance in the healthcare sector and is here to stay. AI applications in healthcare range from AI-assisted robotic surgery to virtual nursing assistants, but also aid clinical judgment or diagnosis, workflow and administrative tasks and image analysis. Great progress has been made in detecting, diagnosing and treating diseases, which contributes to a higher quality of care. AI applications can also contribute to improving the efficiency of providing care and can contribute to reducing healthcare costs.
The growing use of AI in healthcare is associated with an extreme demand for high quality data. Data quality can be defined as the degree to which a given dataset meets a user’s requirements. The quality of data can be assessed by its accuracy, precision, completeness, comprehensiveness, consistency, timeliness, uniqueness, cleanness and coherence. Within healthcare, low quality data can be a threat to patient safety and affect medical decision making when using the data for training algorithms. This is also referred to as “garbage in, garbage out”, which practically means that low quality data leads to low quality outcomes.
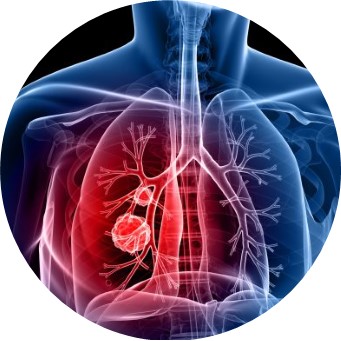
Low quality of data may not be vital in transportation, retail or financial services sectors, but within the healthcare sector it is. AI applications are for example used to determine whether patients have lung cancer. If these applications are trained with low quality data, their outcome is not reliable. Patients with false negative results might not be treated because there seemed no cause for it. In the meantime the cancer can develop further and even become too severe to treat. Situations in which AI based decisions result in life or death should be avoided to guarantee patient safety. To reduce the risk of error prone outcomes, AI algorithms should be trained with high quality data sets.
Considering the growth of AI in healthcare, there is an increasing demand for data. Even with a large demand, the quality of the data should not be compromised. Using low quality data has consequences on the outcomes of algorithms, which can have major adverse effects when applied for medical purposes. Therefore, AI can only be safely implemented if the data that is used for training algorithms is of high quality.
Current data sets are too small
Data sets that are used to train AI algorithms are currently too small to contribute to safe implementation of AI in the healthcare sector. Large amounts of data are needed to train and maintain algorithms and are necessary for AI to thrive. Within the medical industry, healthcare data often consists of hospital records, patients medical records, diagnosis and results of treatment. This highly sensitive personal information asks for strict regulation with regards to owning, processing, analyzing and sharing data. There are many examples of data scandals in which patients rights are violated and patient safety could not be guaranteed. In 2015, a NHS hospital handed over 1.6 million patient records to DeepMind (Google), based on an agreement that lacked patients’ rights and their expectations of privacy. The combination of highly sensitive personal data and data scandals in the past make patients hesitant to share their data for AI related purposes. It is now important to increase patient engagement for sharing data and the development of large data sets.
Current data sets are incomplete

Large data sets are important for initial and ongoing training, validating and improving algorithms. The lack of large amounts makes the outcomes of algorithms unreliable. In addition to data sets being too small, current data sets are often incomplete. Incomplete data sets represent only a part of the population, rather than the whole population. For example, complete datasets should include people with different cultural backgrounds to give an accurate representation of the society. In addition, logical reasoning is not always predictible in medicine. To be precise, one plus one does not always equals two. Patients and their lifestyles are unique, which human care provides are able to understand and interpret in ways AI can not. AI can only draw conclusions based on the data they are fed and are therefore unlikely to consider not obvious solutions. Incomplete data sets, containing not enough diverse patient data, are not appropriate to safely generalize AI outcomes and might therefore be a threat to patient safety.
Current data sets are biased
Current data sets are often biased. This is partly due to the incompleteness of the current databases, for example when they do not include people of every sex, age and orginin. Especially in medicine it is important to have a complete dataset, because of the large number of different causes, diseases and diagnostics. Since biased data also leads to biased output of algorithms, patients can be wrongly diagnosed or treated. Health professionals are concerned about the biased data sets, because biased data sets are not able to take sociodemographics, such as race, ethnicity and gender, into consideration. However, it should be addressed that health professionals themselves contribute to biased data sets as well. This can be caused due to the fact that a doctor unintendedly incorrectly diagnoses a patient and documents this into the electronic health record (EHR). The AI algorithm will often learn from historical EHR data, which implies that the algorithm will make the same mistake as the doctor did earlier. On the contrary, AI can also be an effective tool to help counteract this bias. By strategically deploying AI and carefully selecting underlying data, algorithm developers can mitigate AI bias.
Registration of healthcare data
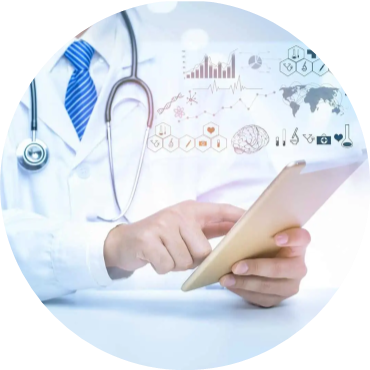
The cause of small, incomplete and biased data sets can be found at the registration of the data. Healthcare data for the development of data sets is often retrieved from EHRs. EHRs are often incomplete and biased themselves, which translate into incompleteness and biased data sets. Healthcare professionals register information about patients, diagnosis, treatment, examination results and medication prescriptions in their own words or by using free-text fields. Free-text fields were initially introduced to provide healthcare professionals flexibility to register their findings. Free-text fields, however, complicate data processing, make data ambiguous and decrease its accuracy. It is therefore important to use registration standards to stimulate the exchange and reuse of data from EHRs. To increase the quality of EHR data, healthcare professionals should be trained and guided to register healthcare data in such a way that it is useful for exchange and reuse.
Differences in standardization and interoperability
Healthcare data, including EHR data, is known for its heterogeneity. Data heterogeneity relates to data with a great variety of different data distributions and complicates its generalization. There are various types of data heterogeneity of which one relates to data standardization and interoperability. Healthcare institutions classify their information by different standards, which results in inconsistency and a lack of interoperability between different institutions. Even when classification standards are being used, valuable data might be lost due to poor data labeling. In the Netherlands, the International Classification of Primary Care is used for coding and classifying symptoms and diseases in the general practitioner’s care. Each of the symptoms and diseases have their own coding. For example, A03 represents fever. However, the codes A97 (no disease) and A99 (other generalized diseases), also called container codes, are often used when general practitioners struggle with diagnosing or when they think that the symptoms or diseases are irrelevant. The A97 and A99 codes lose their relevance for classifying and labeling data, which causes valuable data to be lost.
Differences in population and environment
Another type of heterogeneity that is often seen within healthcare data is population and environment heterogeneity. This type of heterogeneity is caused by differences in patient populations and the environment they are exposed to. Populations might differ in terms of genetics, age, sex and other social demographic characteristics. Populations with a high average age for example, can not be used to train algorithms that are supposed to detect diseases in youth. Differences are also seen in countries where there is an excessive exposure to ultraviolet radiation due to a lot of hours of sunlight. Algorithms to detect melanoma that are trained with data from sunny countries, can not be generalized to countries with less sun. Therefore, high heterogeneous data impedes the generalization of healthcare data from other health institutions, which adversely contributes to the development of large, complete and unbiased data sets.
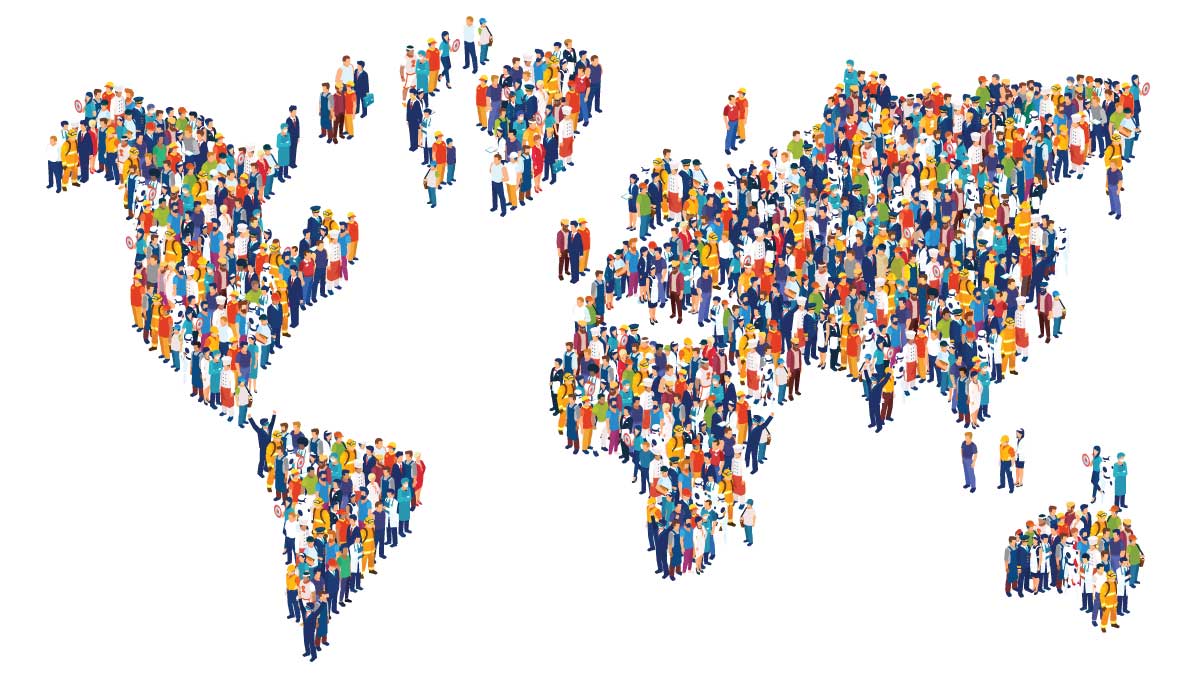
Heterogeneous data can work
However, heterogeneous data might have an advantage. Heterogeneous data sets are needed to build robust data models that can provide precise and personalized care. One example of an application that used large and heterogeneous EHR data is the prediction of the risk of heart failure onset. In this research they validated the accuracy of heart failure risk prediction with a large heterogeneous EHR data set. They concluded that it is possible to generalize recurrent neural network-based deep learning models across hospitals and clinics with different characteristics. However, the accuracy of the recurrent neural network models varied in different patients groups, thus extensive testing is warranted before the implementation of recurrent neural network models in practice.
Already used applications
It is understandable that we have to start somewhere with implementing AI applications in the healthcare sector, since the era of big data is now. We are living in a time in which there are many rapid developments and where everyone wants to be part of the AI revolution. The healthcare sector has already started to use applications like Ezra, which is used to support clinicians in the early detection of cancer. Nowadays, these applications often use small, incomplete or biased data sets, but of course not everything can be perfect from the beginning. It is of utmost importance that we all are aware of the risks that might arise from using AI applications that are trained with low quality data sets. By being aware of the risks, developers can counteract the consequences by taking patients’ safety and ethics into consideration.
Smartwatch data

The development of large data sets is specifically focussed on using EHR data, but private data could be a new source of large amounts of data. For example, data from a smartwatch that measures patients heart rates and physical activity. There is a study that proves that this data can be used to signal physiological changes and underlying illness including change in red blood cells, early signs of dehydration, and anemia. Another way of using this smartwatch data is for identifying atrial fibrillation, a leading cause of a stroke that often goes undiagnosed. The research found that the results highlight the potential role that this data can play in creating more predictive and preventive health care. In addition to the advantages that the use of this data offers, there are also disadvantages from a patients perspective. They have a strong concern for exposing their personal information about their health.
Will the Quality of Data Make or Break AI in Healthcare?
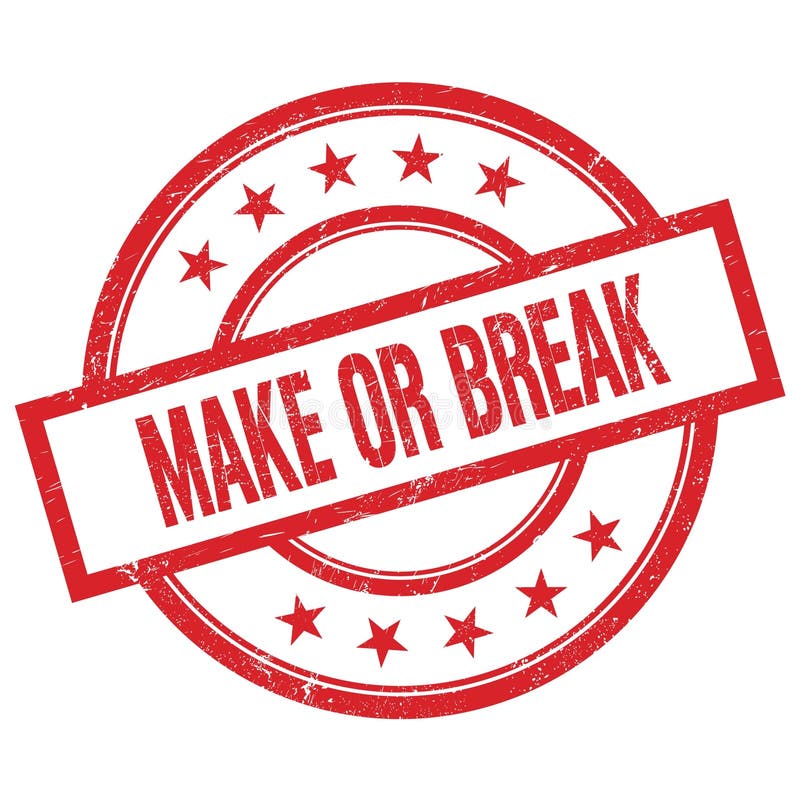
The main question is whether the quality of healthcare data will make or break AI in the healthcare industry. There are currently already various AI applications implemented in the healthcare industry, which contributes to enhanced effectiveness and quality of care. However, the extent to which AI contributes to the improvement of care depends on the quality of the data that is used to train the algorithms. Low quality healthcare data leads to low quality outcomes. When using low quality data sets for medical purposes, patients safety might be endangered. With the growing demand for AI in healthcare, it is of utmost importance to always put patient safety first. In terms of data quality, patient safety can be ensured by training algorithms with data sets of high quality. If patient safety can be ensured, AI can make it in the healthcare sector.