
“How are you doing today?”
“How do you feel?”
These are simple questions that one of your good friends might ask. However, given the rapid advancements of artificial intelligence (AI), it might not be long before we answer these questions to our robot therapists.
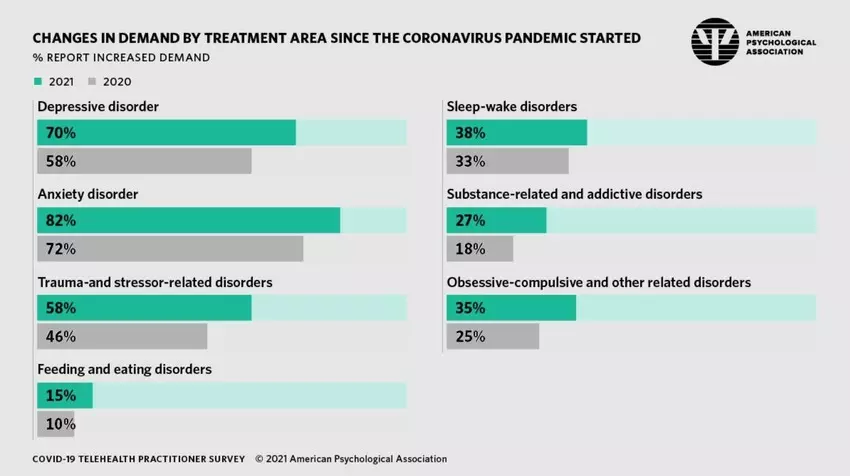
Image: American Psychological Association
The World Health Organization has found that the COVID-19 pandemic has disrupted or halted mental health services in 93% of countries worldwide. At the same time, 84% of psychologists have reported an increase in demand for anxiety treatments since the start of the pandemic. In an attempt to stretch the workload and combat the shortage of mental health services, health professionals are turning to AI for support.
Not only could AI systems provide mental healthcare more easily to those who need it, it could also be a game-changer for supporting therapists in the prediction and diagnosis of mental health problems, and providing treatment that is tailored to the patient’s needs. This is why we argue that AI will help improve the quality of mental healthcare in society. But because the application of AI in mental healthcare is still in its infancy, it is wise to balance its benefits against its limitations.
AI and early prediction of mental health problems
With each passing day, the amount of data that is available about you increases. This data can be used to show you personalized advertisements or give you recommendations to certain posts and videos the algorithm thinks you would like. Some people find this convenient, others would rather not be nudged in their behaviour by their own data. However, this data can also be used for the social good and to monitor online behaviour of individuals that could indicate who is more prone to mental health problems. Your data is then used to develop prediction models for mental health conditions. Prediction is important to catch mental health problems early on and treat them accordingly before they get much worse. Therefore, data-driven AI methods are developed that can accurately and efficiently predict mental health conditions.
To make these prediction models for mental health conditions, a lot of data is needed. More than 6 billion people nowadays own a smartphone, which is a great contributor to obtaining vast amounts of data . This data could be utilized for a method called ‘Digital phenotyping’. Digital phenotyping is a relatively new field of study where data is used to build personalized digital fingerprints of a person’s behaviour and environment. The digital fingerprint can be given as input to machine learning methods that predict mental health conditions of an individual. For example, research showed how a digital fingerprint, in the form of different typing patterns on a touchscreen, could be used to create a classification model that can differentiate between healthy individuals and individuals that have depressive tendencies. Furthermore, research using automated speech-analysis and machine learning was able to correctly separate at-risk young people who developed psychosis over a period of two-and-a-half years and those who did not with 100 percent accuracy.

Methods such as digital phenotyping could be beneficial to solving the capacity problem in mental healthcare. Mental health professionals do not have the resources and time to monitor and predict which of the at-risk people for mental health problems will actually develop them over time. AI prediction models do, they are accurate and efficient, leading to professionals having more time for the treatment of the patients. In order for the precision of digital phenotyping to keep increasing, more data needs to be poured into it. Hence, it is important that individuals trust their smartphone and agree to give their data away as information to mental health models, eventually for their own benefits. To ensure trust we need to make sure that the data collected is not used against the individual and therefore clear governmental frameworks are needed that specify conditions where digital phenotyping is allowed.
More individual diagnoses with AI
If you experience problems with your mental health you seek professional help. In most cases, you have to fill out some questionnaires before your intake assessment so the healthcare professional has a first general idea about which kind of help you need. At the intake assessment, your problems are further elaborated and the professional decides what kind of therapy or medication will work best for you. Unfortunately, it is not often that you find the right treatment straightaway. It can take up to months or even years of trial and error before the right treatment is found. As society we want to aim at finding the right treatment for each individual in the first trial, saving valuable time in the busy schedule of a health professional and increasing the speed of recovery of the patient. AI can be the driving force to obtain this result.
First of all, AI is able to sort through large data sets and uncover relationships that humans just cannot perceive. For instance, if from every individual over the world their psychological questionnaires answers are gathered and their further treatment process is monitored, this will lead to large amounts of data points. If you add the social media activity of the individual or even their genomics to the dataset, it will be too large for humans to make any reasonable sense of it. Deep neural networks (DNN) however are able to integrate many different data sets and provide important insights on how to personalize treatments. Deep neural networks can be seen as a complex transformation machine that can transform a given input into a desired output. In the case of mental health diagnosis, as much data as possible about an individual is given as input and the DNN will give the diagnosis with the possible treatments as output. If this works accurately for every individual, the trial and error phase of the diagnosis process will be eliminated.
Moreover, DNN can improve the efficiency of an intake assessment done by the mental health professional. A DNN can code the conversation between the patient and the professional into a kind of barcode that specifies which subjects were discussed and which techniques were used. After a session the barcode is readily available to be analyzed by the professional. This technique is not yet widely employed but could bring great efficiency benefits in the future. Moreover, a DNN learns and adapts from experience, so the more it is used the better it will perform. And the better the diagnosis process will work, the more rapid a treatment can be started for each individual leading to a hopefully speedy recovery.

Mental health treatment improvements with AI
Treatment types for mental health disorders have changed rapidly over the last decade. Evolution started from conversations held in small settings, to adding teletherapy that allowed to have therapy from a distance. Later, text-based therapy was added and chatbots were created that could perform cognitive behavioural therapy (one of the most common types of psychotherapy). Now, we have the first AI therapist that can perform therapy on her own. The evolution and especially the role of AI in it, provides new effective and convenient ways of revolutionizing mental health therapy.
One of the first revolutions was the addition of teletherapy. Teletherapy can be done by healthcare professionals or AI chatbots. In 2017, Stanford University created a mental health chatbot called Woebot. Woebot implements strategies from cognitive behavioural therapy to help people cope with their anxiety or depressive feelings. A study performed on US college students experiencing symptoms of anxiety and depression showed that after two weeks of using Woebot their symptoms of anxiety and depression significantly reduced. Revealing the potential of AI conversational agents as the new kind of therapists.

The USC institute for creative technologies went one step further and created the first virtual therapist called Ellie. Ellie is able to use computer vision to analyze the patients’ verbal and facial cues and respond supportively. Ellie can perform sympathetic gestures, like nodding or smiling, to let the patient feel at ease and more willing to share his or her own story. What is so special about Ellie is not that she knows how to perform sympathetic gestures, but that she knows when to perform them. Now, you might think that patients find talking to a virtual agent strange and that it might not work that well compared to their human counterpart. However, a study shows the opposite, patients only assessed by Ellie were significantly more likely to open up compared to patients that thought Ellie was directly controlled by a human. Furthermore, the participants felt less judged by Ellie.
Ellie was originally designed to help detect PTSD symptoms of veterans, but shows great potential for other target audiences as well. Important to note is that Ellie is not supposed to completely replace human therapists, but help them in collecting well established baseline data in order to improve treatments. Ellie would be a better first step than her human therapist colleagues, because patients are more willing to open up to her and she can save important information from the session that otherwise could have been overlooked.
AI is vulnerable to societal biases
While AI has the potential to improve the quality of mental healthcare, it is vulnerable to the social and systematic biases that are rooted in society. Healthcare systems can cause unintended harm to patients by propagating these societal biases. This can result in incorrectly diagnosing certain patient groups, like gender and ethnic minorities that are usually underrepresented in datasets.
Algorithmic bias occurs when an algorithm repeatedly produces outputs that are systematically prejudiced, while possibly harming or favoring a group of people. Bias can occur in different ways, i.e. by how data is obtained, how algorithms are designed, and how the outputs are interpreted. The most common cause of bias is by training an algorithm with data that insufficiently represents the target group. For instance, a virtual therapist like Ellie uses computer vision to detect non-verbal cues, such as facial expressions, gestures and eye gaze. If the virtual therapist is largely trained on the faces of tech company employees, it may perform worse in interpreting non-verbal cues from women, people of color or seniors, few of whom work in tech. This could possibly result in inaccurate diagnoses for these people.
How can we eliminate bias in an AI healthcare system? First, it is important to ensure that training data is diverse and represents the whole target group. This can be done by actively including individuals from underrepresented groups that are concerned with the application of the algorithm. These individuals can help identify bias against their communities.
Second, we need to ensure a multidisciplinary cooperation between multiple specialties in the development of algorithms. In this way, AI developers and clinical experts work together to combine the strengths of AI with a deep understanding of mental health and patient care. For instance, the way emotions are felt and expressed vary among populations. This could pose a difficulty for an AI therapist, as it needs to distinguish between different expressions linked to different population groups. In this case, mental health workers can help validate whether algorithmic recommendations are justifiable and not unintendedly harming certain people.
Lastly, fairness metrics need to be applied to thoroughly check developed algorithms. An algorithm may be considered fair if the outcome is independent of sensitive variables, such as gender, ethnicity, or sexual orientation. Investigating the fairness of an algorithm will help to identify the causes of bias and will ensure it performs as intended on the target group.
AI does not experience empathy
Another shortcoming of applying AI in mental healthcare is a system’s limited ability to experience and express empathy. Clinical empathy is the ability to observe and understand a patient’s emotions, and to effectively communicate this understanding to the patient. It is important that mental health practitioners are able to resonate with a patient’s feelings about a particular moment they have experienced. For instance, this moment could be a traumatic event that has caused the patient a lot of anxiety. The ability to express empathy enables more meaningful and effective medical care for at least two reasons.
First, studies show that patients disclose more information about their past if they sense that their therapist visibly resonates with the feelings and thoughts they have shared. It is common that patients do not reveal much information at first. But once they sense emotional resonance during the treatment, they tend to open up more. This could contribute to providing more effective treatment that is tailored to the patient’s needs.
Second, effective medical care depends on how well a patient adheres to the treatment. Studies have found that trust in a therapist is determinative for a patient’s adherence to medical recommendations. If patients sense that their therapist is empathizing with them and genuinely cares for their well-being, they will better adhere to the treatment.
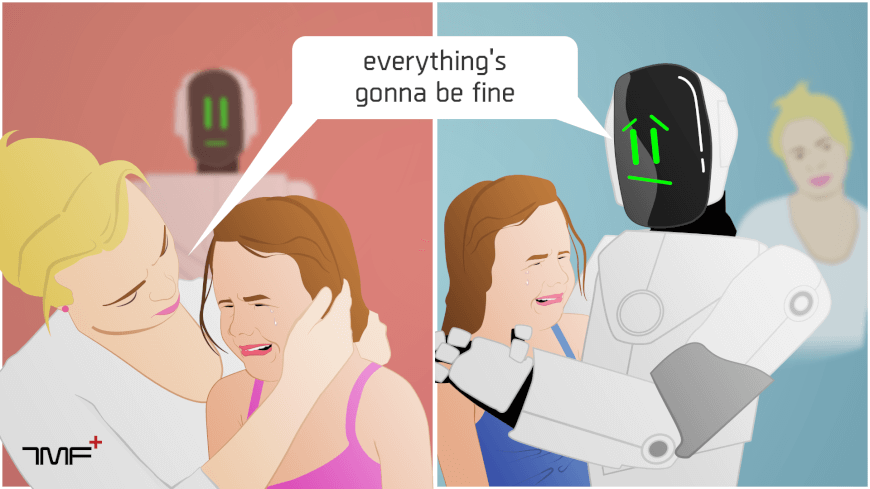
Although AI systems might not experience emotions and empathy the way humans do, artificial empathy, even if not biologically based, might be empathetic enough to provide effective care. As described earlier, empathy is important to let patients disclose information about their past and to improve their adherence to the treatment. These objectives can be achieved even if AI systems do not genuinely experience empathy. It has been found that AI therapists make patients feel more comfortable to share embarrassing events. People tend to disclose more information because they feel they will not be judged by an AI. Moreover, AI systems can improve a patient’s adherence to the treatment by predicting which patients are at risk for nonadherence, identifying necessary actions to prevent nonadherence, and by supporting patients during their treatment.
Researchers argue that it is hard for AI systems to mimic empathy, because the capacities that manifest human empathy are not capacities that an AI therapist can manifest. Humans empathize with each other as a result of the emotions our social brains have evolved through millennia of evolution. We are very good at imagining not only what the world looks like from another person’s perspective, but also what a particular experience must feel like to a person inside. Researchers claim that imagining the feelings experienced by a person cannot be simply reduced to a set of computational rules. AI systems can at most replicate human emotions and respond in the most empathetic way when empathy is called for.
What is next?
Overall, the mental healthcare system requires improvements to keep up with the increasing rise of individuals that experience mental health problems. We have discussed various benefits of AI that could lead to earlier prediction of mental health problems, more individual diagnosis and alternative therapy treatments. However, society has to be cautious and well prepared to enroll AI more into the mental healthcare system. AI is vulnerable for biases and cannot express empathy such as humans do. But these shortcomings of AI are not insurmountable. Different solutions have been proposed to overcome these limitations. What society needs to make sure of now is that the government provides clear frameworks for research and implementation of algorithms used for prediction, diagnosis and treatment of mental healthcare problems. We need to ensure that algorithms are effective and accurate for the majority of people and will only be for the own benefit of individuals. Moreover, if people keep an open mindset into providing their data for the social good, the different algorithms will only increase in accuracy and precision over time. Achieving the full 100 percent accuracy is perhaps not possible, but we believe AI will greatly help improve mental healthcare in society overall.