
The machine plus clinician is better than the clinician, and it’s also better than machine alone
Dr. Eric Topol
Technological changes are often initially treated with scepticism and it can take some time before the benefits become clear and the progress is accepted. This is of course not a problem specific to medicine, but it is often apparent in the history of medicine. For example, when Ignaz Semmelweis first used data and practised evidence-based medicine in 1847/48, concluding that the more frequent deaths of mothers from childbed fever in the Vienna maternity hospital than in private childbirths would be related to poor hygiene standards in the hospital, his findings were rejected by his colleagues throughout his life as “speculative nonsense”. His suggestions to disinfect hands with a chlorine solution and to generally pay more attention to hygiene instead of fumigating the corridors were not only not implemented, but possibly even led to his early death, which circumstances have not been fully clarified until today. A little later, in the beginning of the twentieth century when humanity had already invented cars and aeroplanes, bloodletting was still being discussed in medicine.
Nowadays, of course, medicine has long been scientific, evidence-based, and technologically well-equipped and can no longer be compared to the medicine of yesteryear. However, when it comes to the use of artificial intelligence (AI), development is still in its infancy compared to other fields. Although ideas of computerised medical decision support systems were introduced 50 years ago with good results, the use of AI tools is still very low. For example, a survey showed that only 5% of Dutch doctors use AI. Professor Kohane, head of the Department of Biomedical Informatics at Harvard Medical School, succinctly describes the current state of AI in medicine: “Once again medicine is slow to the mark. I’m no longer irritated but bemused that my kids, in their social sphere, are using more advanced AI than I use in my practice.”
There are manifold reasons for this. First, medical data faces specific privacy requirements, and some diseases are extremely rare, resulting in often limited data sets available. Apart from this, there is also widespread mistrust towards AI in medicine, both on the side of doctors and patients. Moreover, doctors first have to be familiarised with the use of AI systems, as AI is still of little importance in university curricula.
In this article, we demonstrate that the mistrust in AI is unjustified and that AI is able to become an immensely beneficial tool in the health sector. To this end, we highlight many different advantages of AI in medicine, but we also address the problems that arise and the challenges that need to be overcome, and attempt to provide solutions to them.
Current state of AI in health and potential benefits in the future
So let us first take a look into the main current and future applications of AI in the health domain. There are four areas where AI technologies can already be identified: healthcare, health research and drug development, health systems management and planning, and public health and public health surveillance.
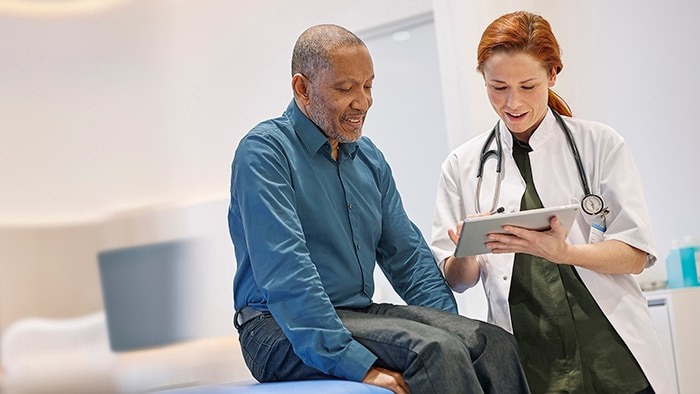
Healthcare. In healthcare, AI is increasingly evaluated as a support tool to improve diagnosis and predictive diagnostics, especially in radiology and medical imaging. For instance, two commercial AI algorithms that identify radiological abnormalities compatible with tuberculosis from X-ray scans were proved to meet WHO’s Target Product Profile minimum criteria for triage tests. According to a recent study, the diagnostic performance of deep learning algorithms in disease detection by medical imaging is comparable to that of healthcare professionals. In fact, their performance is sometimes not only equal but even better. In May 2019, Google and numerous academic medical facilities unveiled a 94% accurate deep learning model developed to diagnose lung cancer, outperforming six radiologists and recording fewer false positives as well as false negatives. When these emergent AI diagnosis systems are validated, medical providers could be able to make faster and more accurate diagnoses.
In addition, AI applications are also increasing in clinical care. Clinicians could use AI to integrate patient records during consultations, identify at-risk and vulnerable patients, support difficult treatment decisions, and detect clinical errors. More importantly, AI is also changing the role of the patient in clinical care. Health monitoring, risk prediction, and conversational agents, so-called chatbots that have implemented the latest improvements in natural language processing, are more and more available in smartphones and wearables. This empowers patients to take more control and self-management, but also more responsibility for their own health. With the COVID 19 pandemic in 2020, this shift from hospital to home care has further accelerated. In China, for example, the number of telehealth providers has almost quadrupled.
Health research and drug development. Another area where AI can lead to major advances is health research and drug development. Important applications of AI in health research include analysing data from electronic health records to identify best clinical practices or develop new ones. In addition, AI is important in genomics, which is the study of an organism’s entire genetic material, as AI could improve human understanding of diseases or identify new biomarkers of diseases.
Furthermore, AI is expected to simplify and accelerate the development of medicines, while making them also cheaper and more effective. DeepMind’s AlphaFold system, which claims to reliably predict the three-dimensional shape of a protein, is an example of a major achievement that is expected to aid in the long process of developing new drugs and vaccines. In view of the predicted advances and optimisations in AI technologies, drug testing could be done virtually in 2040 based on computer models of the human body. Drugs could therefore be tailored to each unique individual.
Health systems management and planning. AI can also assist in the management and administration of health systems and care. Identifying and eliminating fraud or waste, scheduling patients, predicting which patients are unlikely to attend a scheduled appointment, and assisting in the identification of staffing requirements are some of the possible functions of AI in health system management. Additionally, AI is being considered to assist in decision-making in prioritisation or allocation of scarce resources. One example is DeepSOFA, a score framework that uses temporal measurements and interpretable deep learning models to assess illness severity at any point during an ICU stay.
Public health and public health surveillance. AI can also be an important tool for public health. New developments in AI could improve identification of disease outbreaks and support surveillance. For example, AI could be used to promote health, or to identify target populations or locations with “high-risk” behavior that would benefit from health communication via micro targeting. Another application would be to address risks related to environmental or occupational health, such as by analysing air pollution patterns or using machine learning to make inferences between the physical environment and healthy behaviour. In public health surveillance, AI has been applied to build mathematical models based on collected data to to aid decision-making. A recent case was the COVID-19 pandemic, where AI models have been employed to assist in both detection and prediction of regional transmission dynamics, as well as to guide border checks and surveillance.
In summary, the use of AI in healthcare offers enormous potential benefits. In particular, these include more efficient, accurate, and faster diagnoses, better medical care from home, enhanced patient self-management, tailored treatments and better understanding of diseases, improved information processing to detect patterns in data, better prioritisation and allocation of scarce resources, and support for various public health interventions.
From these direct benefits, some indirect advantages may also emerge. By validating AI algorithms and making them accessible worldwide, all the expertise knowledge could be spread. For example, Professor Nicole Wolf, a paediatric neurologist in the Amsterdam Leukodystrophy Center at Amsterdam University Medical Centers (UMC), is specialised in leukodystrophy, a rare genetic white matter disorder, which is only studied in a handful of centres worldwide. In an interview we conducted, she explained that it would be a most welcome help for many centres if the expertise knowledge would be available through AI pattern recognition in MRI changes. Another indirect benefit could arise from the improvement of procedures such as diagnosis tests for patients. For instance, the biomedical engineer Judith Giró won the James Dyson Award in 2020 for her prototype of the Blue Box, a biomedical device for painless, non-irradiating, and low-cost home breast cancer testing. The AI-based algorithm behind it reacts to specific urine metabolites and delivers a classification rate of over 95%. Once this innovative device reaches the market, women around the world will no longer need to undergo to annual irradiating and painful mammograms for breast cancer screening – a test that is often skipped (~40 %), resulting in one third of cancers being detected too late. By making this screening more comfortable and easier, it is likely that the survival rates of women around the world will ultimately improve. There are also other, non health-related improvements by AI in care that need to be highlighted. According to a time and motion observational study of 57 US doctors conducted in 2016, 37% of the time spent with patients involved interacting with a computer screen. Natural language processing, voice recognition, and digital scribing have the potential to reduce the time spent on clerical tasks like writing clinical notes, ordering tests, and prescribing medications from minutes to seconds, leaving more time for patient-doctor interaction.
Challenges that need to be overcome
Despite these tremendous benefits of AI in various medical-related fields, there are of course concerns, risks, and problems associated with the increasing use of artificial intelligence that need to be taken seriously. Which role will physicians play in the future, will they be replaced by robots? How can we ensure that AI systems operate correctly and do not contain biases? Who is accountable in the event of errors? What are the options to ensure security, privacy, and ethical use of sensitive personal data? And can AI really contribute to an improvement in healthcare worldwide?

AI as an assistant for future doctors. Perhaps the biggest problem with the use of AI in medicine is the mistrust it faces. This mistrust is found among both doctors and patients. Among doctors, the growing use of AI raises fears that they will become increasingly dependent on machine assistance in their work and thus neglect their actual work and lose their clinical skills. Some scenarios even paint a future in which human doctors are replaced completely by artificially intelligent robot doctors. However, most experts do not expect this development in the near future. The reasons for this are both the technical limitations of artificially intelligent machines and the patients’ need for direct interaction with human doctors. With regard to the limitations of AI systems, Professor Wolf from the Amsterdam UMC pointed out in the interview that it is difficult to train a computer on highly specialised knowledge, especially with regard to complex symptom combinations of very rare diseases. Therefore, she expects in the medium term, if at all, an automation of the diagnosis. Apart from that, there is a high level of mistrust towards AI systems among patients. Apparently, many feel that their specific problems cannot be adequately addressed by algorithms. Therefore, the need for human interaction and empathy should not be underestimated, which underlines the importance of human doctors.
Instead of replacing doctors, AI systems are expected to augment human skills and to also relieve doctors in many respects, so that the focus can potentially be placed more on the interaction with the patients. As already outlined, AI systems could reduce the long time doctors spend looking at their screens during treatment. In this way, the use of AI would create a win-win situation and possibly also help to increase the acceptance of AI systems on the part of both doctors and patients. Moreover, with modern technology there is sometimes so much data that some doctors urgently desire support and relief in order to cope with the volumes of data. Robert Truog, head of the HMS Center for Bioethics and a pediatric anesthesiologist, emphasises that the volume of data has increased exponentially in the last ten years and that it is impossible for him to consider all the available information. He therefore concludes: “So AI is coming at the perfect time. It has the potential to rescue us from data overload.”
Trustworthy AI. To build trust in AI systems, it is obviously important that they work reliably and correctly. And although, as stated above, this has often been demonstrated, some errors and biases that have been uncovered have sown distrust and cast doubt on the use of AI systems. For example, one study revealed that a widely used system for predicting patients who need special help contained a racial bias. According to this, white people were significantly more likely to receive extra help than black people. A gender bias has also been demonstrated. Similarly, when AI systems are used in the diagnosis of diseases, there are concerns that they do not work equally well for minorities and people from poorer regions of the world. This is because the vast majority of data sets used to train the algorithms come from Europe, North America, and Oceania. This way, existing inequalities in the society are reflected. While some therefore consider the use of such algorithms extremely dangerous, others also see the potential to bring implicit inequalities into the spotlight and make them quantifiable. However, everyone agrees that such biases as well as erroneous decisions should be avoided as far as possible, especially since in the case of flawed algorithms significantly more patients are affected than in the case of a flawed decisions by an individual doctor. To prevent such errors, it is thus very important to ensure diversity of training data and to focus on quality rather than quantity of the data – issues that are already being addressed in the scientific community.
Furthermore, it is crucial to test algorithms under real-life conditions before they are launched and also to regularly re-evaluate them afterwards and correct or even stop them if necessary. In this context, experts emphasise that evaluation should be carried out in an interdisciplinary manner in order to integrate as many different perspectives as possible. Despite all these precautionary measures, errors will most likely occur from time to time, but it must also be considered that the current proportion of human misdiagnoses as well as the associated costs can probably still be drastically reduced.
Shared accountability. Nevertheless, if errors occur, the question of accountability arises. Who is liable when AI algorithms work incorrectly: The treating doctor, the developers, or the company? In order to facilitate AI systems becoming standard practice and to relieve the burden on doctors, it is important that they are not the only ones liable for all possible damages. On the one hand, this is due to the fact that doctors have little or no control over AI systems and their recommendations, and that these systems often make decisions in an opaque manner by means of so-called black box algorithms. On the other hand, it may not be up to individual clinicians to decide in which situations such systems are used. However, doctors should still have a certain degree of liability and the final decision must also be backed by their own expertise in order to prevent an automation bias, according to which only machine instructions are followed mindlessly. In the event of a suspected error, doctors must also be able to act against the recommendations or instructions of an AI system, thereby also providing another safety barrier against flawed algorithms. Ashish Jha, dean of Brown University’s School of Public Health, compares this to the Boeing 737 Max, where the system claimed the plane was going up while the pilots saw it was going down but could not override it. One possibility to support this would be, for example, that several recommendations are made by the machine, from which the doctor should finally choose one. In addition, it would be important that the algorithms used come to decisions that are as transparent as possible. This is also referred to as explainable AI. This is demonstrated, for example, by an AI-based diagnostic system for detecting brain haemorrhages that displays a series of reference images so that a human doctor can check and control the conclusions.
Security and privacy of data. There are also concerns about the privacy and security of sensitive patient data and the fear of hacking. With the increased use of AI systems, the scope of damage from hacking is potentially greater and more personal data is needed for training purposes. If used inappropriately, this data could also contribute to biosurveillance, a form of surveillance in which health data and other biometric characteristics such as facial features, fingerprints, temperature, and pulse are collected. Yet, these are not burning issues that are being created solely through the use of AI, as various ransomware attacks such as WannaCry have proven in the past. Professor Wolf from Amsterdam UMC certainly considers hacking and privacy concerns to be serious, but points out that all patient records are already digital and therefore solutions to these problems have to be found in any case. While increased use of AI may exacerbate the urgency of such solutions, AI monitoring systems, in turn, can also help achieve this safety in healthcare. In addition, concrete measures to protect the particularly sensitive data are already being developed and proposed, such as the decentralised storage of different traninig sets at different institutions and the idea of federated learning, whereby AI models are sent to the different institutions to be trained on their data respectively.
Improve equality of healthcare worldwide. Finally, there is also the question whether AI may be able to reduce or even close the huge global disparities in medical care, or whether it will further increase the existing inequality. Apart from the danger of biases to the disadvantage of already discriminated groups, the question here is especially to what extent such applications will be available worldwide. Because even with bias-free algorithms, the digital divide could ensure that many people and regions with poor infrastructure benefit less. Global efforts are needed to provide the necessary infrastructure worldwide. If this is the case, AI would make it possible to make medical knowledge available all over the world and lead to easier access to medical help even in remote areas. It could also fill the shortage of doctors in poorer countries by enabling individual doctors to care for more people.
A perfect match
Overall, most of the concerns can and must be addressed, so that the benefits of using AI for health clearly outweigh the concerns in our view. The field should set out to realise the tremendous potential in the various areas such as healthcare, health research and drug development, health systems management, and public health. This is also the position of Professor Wolf from the Amsterdam UMC, who anticipates that significant progress in the implementation of AI in medicine will be seen as early as in the next 5 years. In order to contribute to a real improvement in health care worldwide, however, the risks and concerns mentioned above should be taken seriously and, in addition to technical issues, questions of ethics and equality must be placed at the centre.